What cognitive tasks can humans do that AI systems can’t?1 For many years—up until roughly a decade ago—it was easy to answer this question. There are basic tasks that most humans can do with very little effort, but that AI systems weren’t able to do comparably well, such as identifying an object from an image, listening to speech and writing down the corresponding text, and resolving basic linguistic ambiguities.
But over the last few years, it’s been a very different situation. As discussed below in detail, AI systems now match human performance on long-standing benchmarks for image recognition, speech recognition, and language understanding, and while there are questions about reliability of the benchmarks, none of these three broad capabilities are considered major challenges for AI anymore. It now appears difficult to create a durable benchmark at all. Many recently introduced benchmarks have seen AI systems reach parity with humans much faster than expected, including on solving complex math problems posed in natural language and answering challenging questions about biology, physics, and chemistry that take human experts (with access to Google) hours. Many prominent claims about tasks that humans can do and AIs can’t have been quickly falsified, and the practical and commercial applications of AI are quickly growing.
When we ask what cognitive tasks humans can do that AI systems can’t, it is harder today to give a clear answer, much less a stable one. While older benchmarks challenged specialized AIs to compete with the general population of humans, today it’s more common for prominent benchmarks to challenge general-purpose AIs to compete with expert humans on problems that demand both expertise and complex reasoning. This change in the state of benchmarks is unlikely to be a pure measurement artifact, as it has coincided with fast and measurable growth in relevant practical applications for AI.
The difficulty of laying out stable, robust limitations of AI creates a difficulty in policymaking: for almost anything a human can do, there’s a real chance that an AI will be able to do it, and soon. This creates a large space of possible risks—including automated cyber attacks, proliferation of chemical and biological weapons expertise, power imbalances caused by rapid changes in R&D capacity, labor market impacts, and more—that cannot be observed today, but that might be important soon.
It is inherently hard to regulate risks and technologies that do not exist yet. At the same time, waiting for AI risk to become well-characterized and rigorously understood could mean waiting until catastrophic risk has already become too high. It’s not clear how to navigate this challenge, but an important step is recognizing the nature of the challenge—and in particular, recognizing that AI doesn’t have the sort of stable, well-understood limitations it used to.
The rest of this piece will elaborate on the claims above, by:
- Giving examples of well-defined tasks that used to be challenging for AIs, while straightforward for humans
- Summarizing recent progress on AI and attempts to track it with well-defined benchmarks
- Addressing some of the inherent weaknesses of benchmarks as a way of assessing AI capabilities, and discussing other signs that capabilities are improving quickly and impressively
- Discussing some of the key areas where AIs currently struggle to match (expert) human performance
The Past: AI Failed to Match Humans at Basic Tasks
In 1994, the Harvard linguist Steven Pinker wrote:
The main lesson of thirty-five years of AI research is that the hard problems are easy and the easy problems are hard. The mental abilities of a four-year-old that we take for granted—recognizing a face, lifting a pencil, walking across a room, answering a question—in fact solve some of the hardest engineering problems ever conceived.2
This principle is well-illustrated by three basic human skills: image recognition, speech recognition, and basic language understanding.
Image Recognition
In 2010, researchers launched the Large Scale ImageNet Visual Recognition Challenge, in which AI researchers essentially competed to build systems that could accurately identify what sorts of objects were featured in images collected from the internet.3
At that time, the best-performing AI misclassified 28.2 percent of the images it was tested on.4 By contrast, two human annotators achieved much lower error rates of 5.1 percent and 12 percent respectively (and the latter was attributed partly to relative lack of experience with the task).5
AI performance improved greatly with the landmark introduction of AlexNet in 2012 (often credited as kickstarting the current era of high investment in and progress from deep learning). Three years after that, the best AI’s performance exceeded the 95 percent accuracy of the stronger expert human annotator.6
For reasons discussed below, this shouldn’t be interpreted to mean that AIs were fully human-level at vision at that point. But prior to that point, it was much easier to demonstrate their deficiencies.
Speech Recognition
A prominent benchmark for speech recognition is the Switchboard Hub 5’00 dataset,7 with recorded phone conversations that AIs or humans can transcribe into text. The benchmark was introduced in 2000; as late as 2011, state-of-the-art AI performance still had error rates of over 15 percent (see Table 1 here for example), compared to 5–6 percent for humans.8 The gap was closed in 2016–2017.9
Basic Language Understanding
A number of different benchmarks have been designed for natural language understanding. Here I’ll focus on one that is relatively simple, long-standing, and was originally proposed as an “alternative to the Turing Test:” Winograd schemas. These challenge an AI (or human) to answer questions—simple for humans, but (previously) challenging for AI—like:
The trophy doesn’t fit in the brown suitcase because it’s too big. What is too big?
Answer 0: the trophy
Answer 1: the suitcase
Joan made sure to thank Susan for all the help she had given. Who had given the help?
Answer 0: Joan
Answer 1: Susan
Note that simple substitutions, such as replacing “big” with “small” in the first example or “given” with “received” in the second, would change the answers. The paper introducing the Winograd Schema Challenge argues that, therefore, “clever tricks involving word order or other features of words . . . will not work. . . . Doing better than guessing requires subjects to figure out what is going on.”10
The Winograd Schema Challenge and associated datasets were introduced in 2010–2011.11 In 2016, a cash prize was offered for AI systems that could achieve 90 percent accuracy (roughly in line with human performance12), but the best entrants not only fell well short, they barely outperformed chance—as discussed in an MIT Technology Review article called “Tougher Turing Test Exposes Chatbots’ Stupidity.”13
By 2019, 90 percent accuracy had been achieved, and there were concerns that this was being done via “tricks”—exploiting biases in the dataset—rather than via AI achieving “common sense reasoning.”14 A new dataset, WinoGrande, was created to address this issue, filtering specifically for problems similar to those above that could not be solved by particular sorts of brute force pattern-matching techniques.15 At the time of release, humans had 94 percent accuracy on this dataset, compared to 59-74 percent for the “best state-of-the-art [AI] methods.”16
A 2022 language model achieved 96.1 percent accuracy on WinoGrande (with limited dataset-specific optimizations).17 GPT-4, released in 2023, achieved 87.5 percent accuracy with almost no WinoGrande-specific optimization.18
Table 1: Progress on Basic AI Capabilities | |||
Capability | Dataset/Challenge | Year Established | Year of AI Surpassing Human Performance |
Image recognition | Large Scale ImageNet Visual Recognition Challenge | 2010 (though similar datasets had existed for years previously) | 2015 |
Speech recognition | Switchboard Hub 5’00 | 2000 | 2017 |
Resolving basic language ambiguities | Winograd Schema Challenge and WinoGrande | Dataset introduced 2010–2011; cash prize unclaimed in 2016; new, harder dataset introduced in 2019 | 2022 |
As discussed below, there could be significant measurement issues here. Human-level performance may have been achieved through intense specialization and optimization for the benchmark at hand or could otherwise reflect deficiencies in the benchmarks.
However—speaking informally, as this is not the kind of claim that is amenable to rigorous evidence—none of these three broad capabilities are considered major challenges for AI anymore, and today’s large-language-model-based products appear to be generally quite competent at all three. The reader can confirm the latter with tests of their own (upload an image and ask the model to discuss the image; interact with the model in voice mode; ask it to solve a Winograd-schema-like challenge).
Recent Progress and Evolving Benchmarks
In more recent years, benchmarks for AIs have been on more challenging tasks—and have been much shorter-lived. A chart from Stanford’s most recent AI Index Report illustrates this:
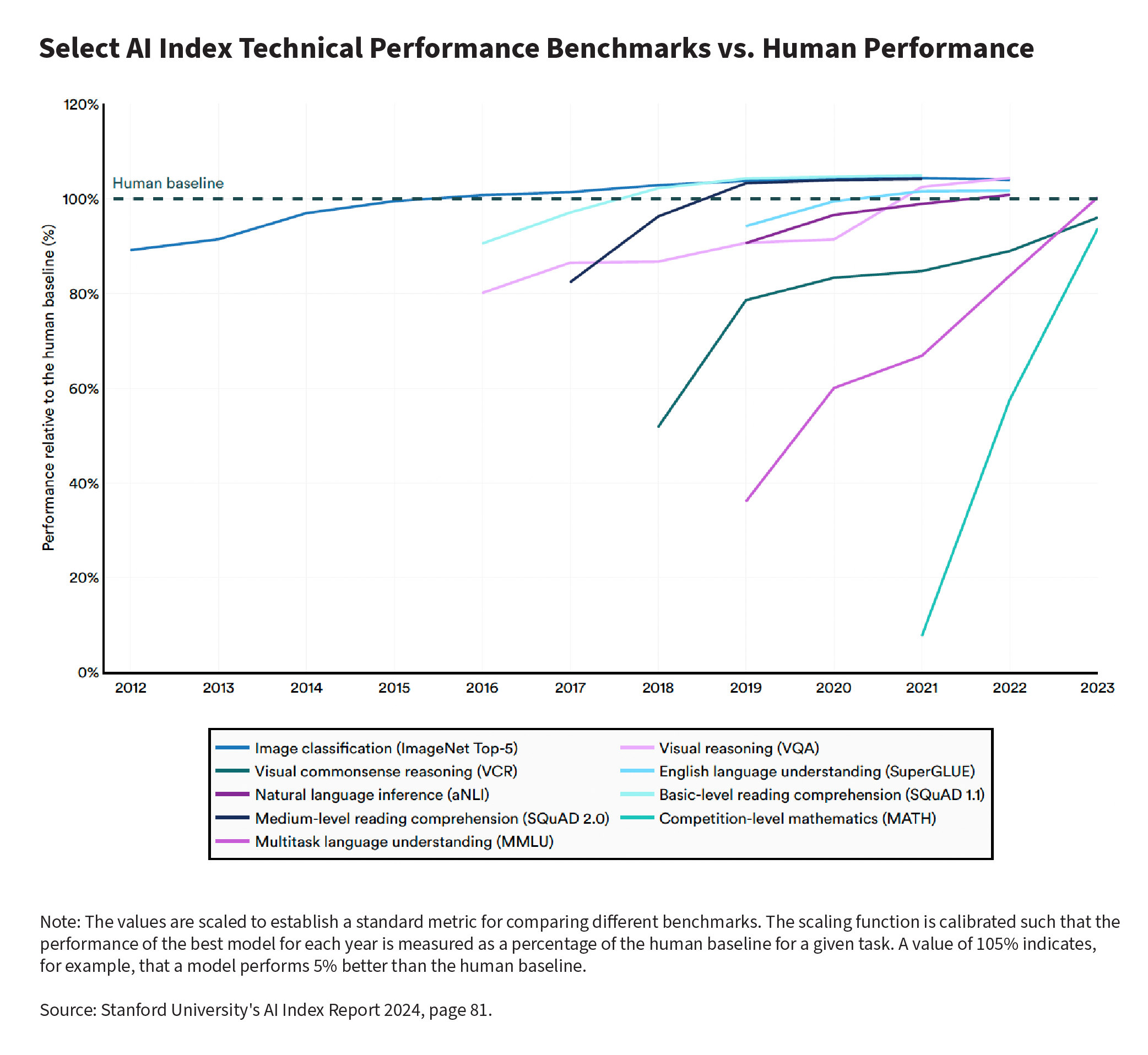
Each line represents state-of-the-art AI performance on some published benchmark. The chart shows that performance has been reaching human level faster and faster as new benchmarks have been created.
And many of these benchmarks were designed specifically to capture things that the authors thought would stay difficult for AI for a long time. For example, the paper introducing the SuperGLUE benchmark (for language understanding) featured an intention to “take into account the lessons learnt from [the] original GLUE benchmark,” which became obsolete a year after it was introduced in 2019 due to AI approaching human-level performance.19 Yet SuperGLUE itself saw the same fate (AIs exceeding the human-level benchmark) only two years after it was introduced.20
Another example: in the paper introducing the MATH benchmark (competition mathematics problems), the authors predicted that the current AI paradigm would not achieve human-level performance, and that new types of breakthroughs would be needed.21 Two examples of MATH problems:
Problem: Suppose a and b are positive numbers with a > b and ab = 8. Find the minimum value of (a2 + b2) / (a-b)
Problem: Right ΔABC has legs measuring 8 cm and 15 cm. The triangle is rotated about one of its legs. What is the number of cubic centimeters in the maximum possible volume of the resulting solid? Express your answer in terms of π.
Yet the accuracy of AI systems on problems like these shot up very shortly after the benchmark was introduced, with only minor adjustments to the ways AI systems were being trained and set up for the benchmark.22 This was wildly outside the most aggressive predictions of a group of specialized forecasters who had registered their predictions as part of a contest.
Broadly similar dynamics apply to several of the other benchmarks, as well to some not listed. For example, consider GPQA,
a challenging dataset of 448 multiple-choice questions written by domain experts in biology, physics, and chemistry. We ensure that the questions are high-quality and extremely difficult: experts who have or are pursuing PhDs in the corresponding domains reach 65% accuracy (74% when discounting clear mistakes the experts identified in retrospect), while highly skilled non-expert validators only reach 34% accuracy, despite spending on average over 30 minutes with unrestricted access to the web (i.e., the questions are ‘Google-proof’).
An AI achieved a score of 59.5 percent on this benchmark—very close to the human expert score—only four months after GPQA was published. Less than three months after that, OpenAI’s o1 model solidly beat the human expert score.
Benchmarks Don’t Tell the Whole Story
This piece has focused on formal benchmarks: large sets of standardized tasks that AIs can be challenged to complete. Benchmarks provide a relatively systematic, objective look at trends in AI performance over time. But there are limitations to what benchmarks can establish about AI capabilities.
Any given benchmark might have design issues that make it easier for AI systems than intended, and/or harder for humans. For example, see Melanie Mitchell’s critique of the WinoGrande benchmark, which raises concerns about the quality of the sentences that AI systems are tested on (since they were assembled in vast quantities using Mechanical Turk), as well as about whether the methods used for filtering out overly AI-friendly challenges were unreliable.23
Additionally, benchmarks might often be designed to be neither easy nor out of reach for the AI systems of the time, since overly easy and overly difficult benchmarks are less likely to generate interesting results. Benchmarks designed along these lines are inherently likely to see major progress and even saturation as AI systems improve, and they might not be measuring the full capabilities that humans have in the domain in question. For example, the image recognition challenge discussed above featured well-lit images with an obvious subject; AIs matching humans on this benchmark can’t necessarily match humans in other vision tasks, such as dealing with poor lighting.
Another note about benchmarks is that defining “human-level performance” is not necessarily straightforward, and in many cases it is done using very rough methods. For example, the ImageNet paper discussed above based its estimate of human performance on only two humans, and did not give detailed criteria for how they were chosen.24
But even with these caveats in mind, it’s striking how much the dynamics of benchmarks have changed in the last decade or so. In the past, simple and straightforward tasks that are easy for nearly all humans proved to be strong challenges for AI systems for years; in more recent years, much more difficult benchmarks have been obsoleted with striking (and accelerating) speed. And the picture painted here is consistent with other observations about accelerating AI progress.
One such observation: there have recently been a number of instances of people prominently announcing challenges or tasks AI systems can’t do, only for general-purpose AI systems to succeed very quickly afterward. For example, one tech entrepreneur offered $10,000 for anyone that could get a general-purpose language model to complete a specific task he had designed as a test of “long-term reasoning” and “truly learn[ing] new problems”; the money was claimed two days later. In early 2023, an economics professor publicly bet that no AI would reliably score an A on his economics exam before 2029; yet three months later, he conceded: “To my surprise and no small dismay, GPT-4 got an A. It earned 73/100, which would have been the fourth-highest score on the test.”
And there are a number of other signs that the story of AI acceleration told by benchmarks is a real one. One of these is the sharp increase in the practical and commercial usefulness of AI systems. A 2024 NBER working paper states that “Historical data on usage and mass-market product launches suggest that U.S. adoption of generative AI has been faster than adoption of the personal computer and the internet.” A 2024 article from McKinsey details trends in adoption, including that “65 percent of respondents report that their organizations are regularly using [generative] AI, nearly double the percentage from our previous survey just ten months ago.” Recent studies suggest that AI can have very large impacts on productivity for coders, consultants, and even materials science researchers. Driverless taxis have recently become a reality, suggesting that AI vision has gone well beyond labeling clear images.
What Are the Open Challenges for AI Models Today?
Stanford’s most recent AI Index Report catalogues performance on twenty-one different benchmarks for AI. Of these, AI is already at or near human-level performance on eight. For another ten of them, no human benchmark appears to be available for a number of reasons, including recency (tests introduced in the previous year) and the potential impracticality of human benchmarks (for example, video and image generation, or tests based on asking which of two models a human user finds more useful).
That leaves three benchmarks where AI systems still seem to lag human performance significantly. Two of these, HaluEval and TruthfulQA, specifically target AI systems’ tendency to “hallucinate” or fabricate answers. The third, SWE-bench, assesses AIs’ ability to autonomously resolve real-world issues via software engineering. These reflect two larger themes for today’s benchmarks.
Idiosyncratic Weaknesses
While impressive in some ways,25 today’s large language models demonstrate idiosyncratic and sometimes strange weaknesses. The tendency to hallucinate is one. Another weakness: AI systems sometimes struggle to adapt to seemingly minor or irrelevant changes in questions (for example, being able to correctly answer “Who is Tom Cruise’s mother? [Mary Lee Pfeiffer]” but not “Who is Mary Lee Pfeiffer’s son?”)26 Relatedly, a recent paper shows AI systems’ performance on math problems degrading when numbers are changed around and (especially) when additional clauses are added to questions. Some simple, vivid shortcomings have become widely known, such as incorrectly counting the R’s in the word strawberry or losing at tic-tac-toe.27
Real-World Applications
SWE-bench consists of “2,294 software engineering problems drawn from real GitHub issues. . . . Given a codebase along with a description of an issue to be resolved, a language model is tasked with editing the codebase to address the issue.”28 This is essentially asking whether an AI system can complete tasks typically associated not with basic understanding so much as value creation—of the kind typically done by skilled human software engineers. In order to test language models on such tasks, evaluators create “agent scaffolding” that gives the models the ability to do things like “run code on a computer, read and write files, browse the internet and otherwise interact with the world in a similar way to a human could from a text-only command line interface.”29
The AI Index Report documents very poor performance—no model, even “assisted,” resolved more than 5 percent of the issues on SWE-bench. As of this writing about a year later, the best score is 22 percent,30 and questions have also been raised about the dataset’s quality, so an improved smaller dataset—SWE-bench Verified—has been introduced, with the best AI systems able to resolve just over half of the issues.31
Since the AI Index Report was published, there have been further benchmarks created and further impressive progress. For example, the extremely challenging FrontierMath benchmark has been created, and state-of-the-art performance on it has improved from 2 percent of problems solved to around 25 percent.
Another category of evaluations comes from the growing interest in assessing AI models’ capability to cause harm (either autonomously or by assisting harmful actors). Most major releases of large language models have been accompanied by “model cards” or “system cards” reporting on attempts to assess whether AI models can, for example, deceive and manipulate human operators, find and exploit cybersecurity vulnerabilities, autonomously carry out complex software engineering projects (SWE-bench is one dataset used for this, but not the only one),32 and “help experts with the operational planning of reproducing a known biological threat.”33 For many such tasks, AIs still do not appear to be on par with human experts, but are becoming more capable.34
If a Human Can Do It . . .
It’s become very difficult to confidently lay out a challenge that AI models consistently fail and will likely continue to fail for years to come. If we can define a task that a human, even a human expert, can complete digitally (that is, by using interfaces that are available to AIs without needing a physical presence, a particular legal status, and so on), the odds are good that an AI may be able to do it too sometime in the coming years.
Sufficiently powerful models could pose serious risks to international security—especially if the models become widely distributed, have access to significant resources, and can interact with a large number of actors, some malicious. A previous piece goes through several examples, including proliferation of the ability to produce weapons of mass destruction; the ability to automate cyber attacks; cost-effective, scalable political persuasion interventions; and the potential to automate research and development work, leading to rapid progress in fields including robotics, weaponry, and AI itself.
This creates a challenge for policymakers. Typically, risk management involves addressing relatively well-understood risks with proven or familiar procedures. When it comes to AI, there’s a large surface area of potential threats that may or may not emerge in the near future.
This article has focused on documenting the fast, disorienting progress of AI capabilities that creates this challenge. It has not focused on how policymakers can deal with this challenge. Previous pieces have laid out some ideas for doing so:
- One discusses if-then commitments as an approach to mitigating future, unknown risks without unnecessarily slowing the development of new technology.
- Another proposes a general philosophy of trying to develop risk management practices via early release and iteration (a similar approach to how AI companies tend to develop their products), rather than via perfectionist up-front research.
Whether or not these sorts of ideas prove workable, policymakers need some way of contending with a dynamic and unpredictable landscape of potential catastrophic risks. It’s inherently hard to responsibly regulate and prepare for technologies that don’t exist yet, but in the case of AI, it may be necessary.
Thanks to Lawrence Chan, Ajeya Cotra, and Max Nadeau for comments on a draft.
Notes
1This piece focuses on cognitive tasks, rather than asking the broader question “What can a human do that an AI can’t?” The latter could include physical activities (robotics progress does not have the same qualities that this piece attributes to AI progress), social interaction, and so on.
2Steven Pinker, The Language Instinct (William Morrow and Co., 1994).
3See page 12 of ImageNet Large Scale Visual Recognition Challenge for sample images from the dataset.
4See page 19 of ImageNet Large Scale Visual Recognition Challenge. The specific metric used was “top-5 classification error,” which means the AI listed five classes that the object in the image might belong to, and it was classified as an error if none of the five matched the image.
5From pages 31–33 of ImageNet Large Scale Visual Recognition Challenge: “Annotator A1 evaluated a total of 1500 test set images. . . . The human error was estimated to be 5.1%. . . . Our second annotator (A2) trained on a smaller sample of only 100 images and then labeled 258 test set images. As seen in Table 9, the final classification error is significantly worse, at approximately 12.0% Top-5 error. The majority of these errors (48.8%) can be attributed to the annotator failing to spot and consider the ground truth label as an option.
“Thus, we conclude that a significant amount of training time is necessary for a human to achieve competitive performance. . . . The annotator may sometimes be unaware of the ground truth class present as a label option. When pointed out as an ILSVRC class, it is usually clear that the label applies to the image. These errors get progressively less frequent as the annotator becomes more familiar with ILSVRC classes.”
6See page 47 of the Artificial Intelligence Index 2018 Annual Report.
7This is the only speech recognition dataset featured or referenced in the Stanford AI Index Report prior to the 2022 edition; it is also used in Kiela et al. 2021, which summarizes AI progress on a number of benchmarks. More information about the dataset is available here.
8A 2016 paper examines the human benchmark.
9See page 61 of the AI Index 2018 Annual Report.
10Later papers would question this claim, for example The Defeat of the Winograd Schema Challenge and WinoGrande: An Adversarial Winograd Schema Challenge at Scale.
11From a Collection of Winograd Schemas: “Building on Levesque’s initial work, in 2010, Ernest Davis created an additional eighty-nine schemas. Together with four suggested by Ray Jackendoff, these were published on the web in HTML and XML.”
12See table A.2 in The Defeat of the Winograd Schema Challenge.
13Tougher Turing Test Exposes Chatbots’ Stupidity: “The programs entered into the challenge were a little better than random at choosing the correct meaning of sentences. The best two entrants were correct 48 percent of the time, compared to 45 percent if the answers are chosen at random. To be eligible to claim the grand prize of $25,000, entrants would need to achieve at least 90 percent accuracy.”
14See WinoGrande: An Adversarial Winograd Schema Challenge at Scale, the paper introducing the WinoGrande benchmark discussed below.
15See page 3 of WinoGrande: An Adversarial Winograd Schema Challenge at Scale.
16See the abstract of WinoGrande: An Adversarial Winograd Schema Challenge at Scale.
17ST-MoE: Designing Stable and Transferable Sparse Expert Models (see table 12). The model was general-purpose, but fine-tuned on the WinoGrande task.
18The GPT-4 announcement says the performance was achieved "5-shot" (see second table), which means essentially that the language model was given 5 examples of questions and correct answers before being tested (more explanation here).
19See the SuperGLUE website and paper.
20See page 100 of the 2023 AI Index report.
21From the paper introducing the MATH benchmark: “We find that simply increasing budgets and model parameter counts will be impractical for achieving strong mathematical reasoning if scaling trends continue. While scaling Transformers is automatically solving most other text-based tasks, scaling is not currently solving MATH. To have more traction on mathematical problem solving we will likely need new algorithmic advancements from the broader research community.”
22A July 2022 paper, Solving Quantitative Reasoning Problems with Language Models, goes into detail on the improvements that were made to achieve strong performance. A key quote (though other relatively minor adjustments were made as well): “The main novelty of this paper is a large training dataset that juxtaposes natural language with the correct use of formal mathematical language, such as equations and diagrams.”
23From Mitchell's column, What Does It Mean for AI to Understand?: “The WinoGrande results come with some important caveats. For example, because the sentences relied on Amazon Mechanical Turk workers, the quality and coherence of the writing is quite uneven. Also, the 'unsophisticated' AI method used to weed out 'non-Google-proof' sentences may have been too unsophisticated to spot all possible statistical shortcuts available to a huge neural network, and it only applied to individual sentences, so some of the remaining sentences ended up losing their ‘twin.’ One follow-up study showed that neural network language models tested on twin sentences only—and required to be correct on both—are much less accurate than humans, showing that the earlier 90% result is less significant than it seemed.”
24See Section 6.4.1. on page 31 of ImageNet Large Scale Visual Recognition Challenge.
25For example, see the discussion of GPQA above.
27Caveat: while writing this piece, I tried both of these challenges on two AI systems; one got both correct and the other got one correct. This may reflect further recent progress, or may reflect that the issues noted in the main text are not consistently present.
28See SWE-bench: Can Language Models Resolve Real-World GitHub Issues? This is the paper that introduced SWE-bench.
29See METR's mid-2023 report on model capabilities for an example.
30From the leaderboard at SWE-bench at the time of writing (November 24, 2024).
31From the leaderboard at SWE-bench at the time of writing (November 24, 2024).
32Other examples include METR's task suites and MLE-Bench.
33For example, see the OpenAI o1 System Card. Most major large language model releases are accompanied by a “model card” or “system card” in this general vein, though often less thorough.
34See discussions in the OpenAI o1 System Card.