Introduction
In the last few years, as OpenAI’s ChatGPT, Anthropic’s Claude, Google’s Gemini, and Meta’s LLaMA captured the world’s imagination with the possibilities of large language models (LLMs), several Southeast Asian LLMs emerged from the shadows to specifically foreground regional languages and contexts.
In December 2023, AI Singapore’s South East Asian Languages in One Network (SEA-LION) and Alibaba DAMO Academy’s SeaLLM debuted within days of each other. Both developers released a similar suite of regional languages, including Burmese, Indonesian, Khmer, Lao, Malay, Thai, Vietnamese, as well as English and Chinese. A few months later, in April 2024, SEA AI Lab and the Singapore University of Technology and Design introduced Sailor, comprising English, Chinese, Vietnamese, Thai, Indonesian, Malay, and Lao.1 SEA AI Lab is a part of SEA Limited, a consumer internet company founded in Singapore that operates digital entertainment, e-commerce, as well as digital payments and financial services businesses. As region-wide LLMs, SEA-LION, SeaLLM, and Sailor have been paralleled and, in some cases, preceded by the development of other models focused on local languages spoken in Indonesia, Thailand, and Vietnam (see table 1).
In riding the wave of LLMs, Southeast Asian developers have recognized the enormous opportunity to “democratize access to advanced language technologies for regional and potentially under-represented languages.”2 By plugging the regional gap on what natural language processing (NLP) researchers term “low resource languages”—in other words, languages with limited high-quality, well-labeled data that may also be hampered by a lack of funding, infrastructure, or linguistic expertise—developers have consciously sought to depart from “the western, industrialized, rich, educated, and democratic (WIRED) basis of these models.”3
With 40 percent of LLMs today produced by U.S.-based companies—many trained on the English language, with at least one company, OpenAI, self-reporting a Western- and English-language bias4—these Southeast Asian models are an apparent expression of agency in an ecosystem otherwise dominated by the languages, worldviews, and resources of others.5
But Southeast Asia is an incredibly diverse region with an ancient civilizational history that is, today, a collection of young postcolonial nation-states in search of a reimagined identity. With over 1,200 languages spoken, language is deeply political.6 In Indonesia, alone, more than 700 languages are spoken among the nation’s 300 million–strong population, making the country the second-most linguistically diverse after Papua New Guinea.7 Even though Cambodia is approximately seventeen times smaller than Indonesia, an estimated twenty-seven languages are spoken among its population of 17 million people.8
Far from its utilitarian function as a tool of communication, language as a distinct marker of identity encodes cultural values, traditions, and knowledge perspectives. Because language is profoundly personal and communal, it has been repeatedly used by those in power to unite a nation or divide a society.
This paper explores how the development of Southeast Asia–specific LLMs intersects with the politics and particularities of languages in the region. This relationship, in turn, shapes Southeast Asia’s notions of representation and agency on the global stage of artificial intelligence (AI). By examining how advanced language technology and the broader social context are co-dependent and mutually reinforcing, this paper takes a sociotechnical and cross-disciplinary approach to understanding how Southeast Asian developers are filling the low resource language gap in existing LLMs.9 Models can only be effectively trained and deployed for use by society when there are data drawn from experiences and interactions among human beings as well as between humans and their surroundings. Fundamentally, this paper explores the social underpinnings and implications of initiatives often taken for granted as purely technical endeavors.
The first section offers an overview of LLMs in Southeast Asia. It outlines the rationale for these models, the models’ developers, and the architectural framework, as well as how much the models have grown in only the last few years. The second section contextualizes the complex sociopolitical landscape of these regional LLMs by outlining how language policies have evolved in countries across Southeast Asia, driven by domestic objectives and global realities. The consolidation of national identities in many of these countries, achieved by language policies coupled with greater economic prosperity through industrialization, paved the way for the region’s growing self-awareness and confidence on the world stage. This unfolding self-actualization, in turn, has influenced more recent aspirations for greater representation and agency in the digital space as governments and other stakeholders customize tech products and services developed for the masses or build their own. Homegrown LLMs are a perfect example of both.
The third section identifies the opportunities presented by these local LLMs, while the fourth section addresses their wider challenges. The analysis reveals several important conclusions: There is growing and actionable resolve by AI researchers in Southeast Asia to redress the lack of representation of the region’s languages and worldviews in Western-led LLM development. This is most evident in the number of models in the region that have been released in just the last four years, driven by the technical community’s shared motivation to innovate for the region’s particularities. This burgeoning confidence about Southeast Asia’s place in the world is a nascent but promising step for the region to cultivate technological agency, capability, and skills in the strategic realm. Despite this robust trajectory, however, there is little in-depth, cross-fertilization of perspectives among the technical and nontechnical communities in Southeast Asia on how to faithfully and responsibly represent the region’s linguistic complexities and cultural nuances while navigating political sensitivities associated with language. This gap presents an opportunity for developers, practitioners, academics, and civil society to engage more creatively with counterparts within and beyond the region—particularly, in the “majority world” or what is broadly described as the Global South—on issues impacting low resource language model development.10 Accordingly, the fifth section proposes a set of policy recommendations for various Southeast Asian stakeholders to consider as developers build or fine-tune existing LLMs.
At the end of this paper, we include a glossary of technical terms frequently associated with LLMs, for the nontechnical reader. We also append several annexes: Two comprehensive tables of different LLM initiatives and benchmarks in Southeast Asia that are, nonetheless, still non-exhaustive. There were updated versions of existing models or new models being released even as we were finalizing this paper in December 2024. Finally, we also republish the results of a simple prompt experiment we ran on ChatGPT and SeaLLM’s chat function, comparing their responses in English, Malay, and Thai.
An Overview of Southeast Asia–Focused LLMs
Prior to 2020, Southeast Asia’s technical community began seeding the regional ground for language model development in the areas of machine translation, voice recognition, and sentiment analysis in local languages. In the case of sentiment analysis, these efforts were primarily for research, commercial, and even political purposes.11 In the background, governments, keenly cognizant of the transformative potential of data-driven technologies, began developing national AI strategies to foster ecosystems ripe for local innovation and foreign investment.12
Between 2020 and 2022, researchers released the first small wave of Southeast Asian–focused language model initiatives. These were limited in the number of languages, scope, and performance, but the technical community’s efforts at benchmarking and resource collection were a step toward more ambitious goals (see Annexes 1 and 2).
The release of ChatGPT in November 2023 has been paralleled by a rapid increase in LLM projects in countries such as Indonesia, Singapore, Thailand, and Vietnam. Apart from the multilingual regional initiatives of SEA-LION, SeaLLM, and Sailor mentioned above, monolingual models such as Indonesia’s IndoBERT, Malaysia’s MaLLaM, Thailand’s OpenThaiGPT, and Vietnam’s PhoBERT have also made their appearance. Academic, enterprise, and government stakeholders—often with the partnership or support of multinational companies—understand the profound importance and commercial potential of localizing generative AI tools for greater use and access.
Data collated by AI Singapore from Hugging Face, a collaborative platform for the machine-learning community, indicates that 73 percent of existing LLMs originate from the United States and China, and 95 percent of all models are primarily trained on either English-language data or a combination of English and Arabic, Chinese, or Japanese.13 Other research has revealed that 88 percent of the world’s languages are insufficiently represented on the internet, resulting in 20 percent of the world’s more than 1 billion people not being able to use their language to participate in the digital world.14
There has been some progress, however. Released in 2019, Multilingual BERT (M-BERT) was pre-trained on 104 languages, including Indonesian, Javanese, Malay, Minangkabau, Sundanese, and Vietnamese.15 ChatGPT’s range of Southeast Asian languages is less extensive but includes Indonesian, Malay, Thai, and Vietnamese.16
Despite the expansion by frontier LLMs to include regional languages, these advanced models still inadequately capture the precision, subtleties, and cultural context of the region’s tongues.17 When researchers asked ChatGPT to translate thirty sentences, the model correctly rendered twenty-eight from Indonesian to English but only nineteen from English to Indonesian. ChatGPT failed to correctly translate any of the thirty sentences from English to Sundanese, though it did accurately translate nine out of the thirty sentences from Sundanese to English.18
Researchers recognize how far English-based models have advanced in translating and understanding underrepresented regional languages but lament the lingering English-centricity and bias of these larger foundational models. They also perform poorly in code-switching within or between Southeast Asian languages.19 Additionally, at least one study suggests that multilingual LLMs initially “understand queries by converting multilingual inputs into English, think in English in intermediate layers while incorporating multilingual knowledge, and generate responses aligned with the original language in the final layers.”20
Southeast Asia’s technical community has, in earnest, taken up the challenge of plugging this regional representational gap in LLMs. It does this to optimize on-the-ground AI solutions, whether in the form of chatbots or educational tools, in a way that comports best with local expectations rather than compete with the likes of ChatGPT. The region’s NLP researchers are also pushing their own boundaries in this emergent space simply because the science is cool.21 They are keenly aware that, done right, localized LLMs could go a long way in portraying and preserving the region’s rich, cultural fabric more effectively to Southeast Asia’s own populace. In the longer term, a more heightened sense of self-awareness could, in turn, boost nations’ image, confidence, and agency on the international stage.
Between 2020 and 2024, researchers released a total of thirty-five LLMs specific to the region (see figure 1), a remarkable number in that timeframe but one that’s not wholly surprising given the enormous commercial and research potential presented by localized LLMs.
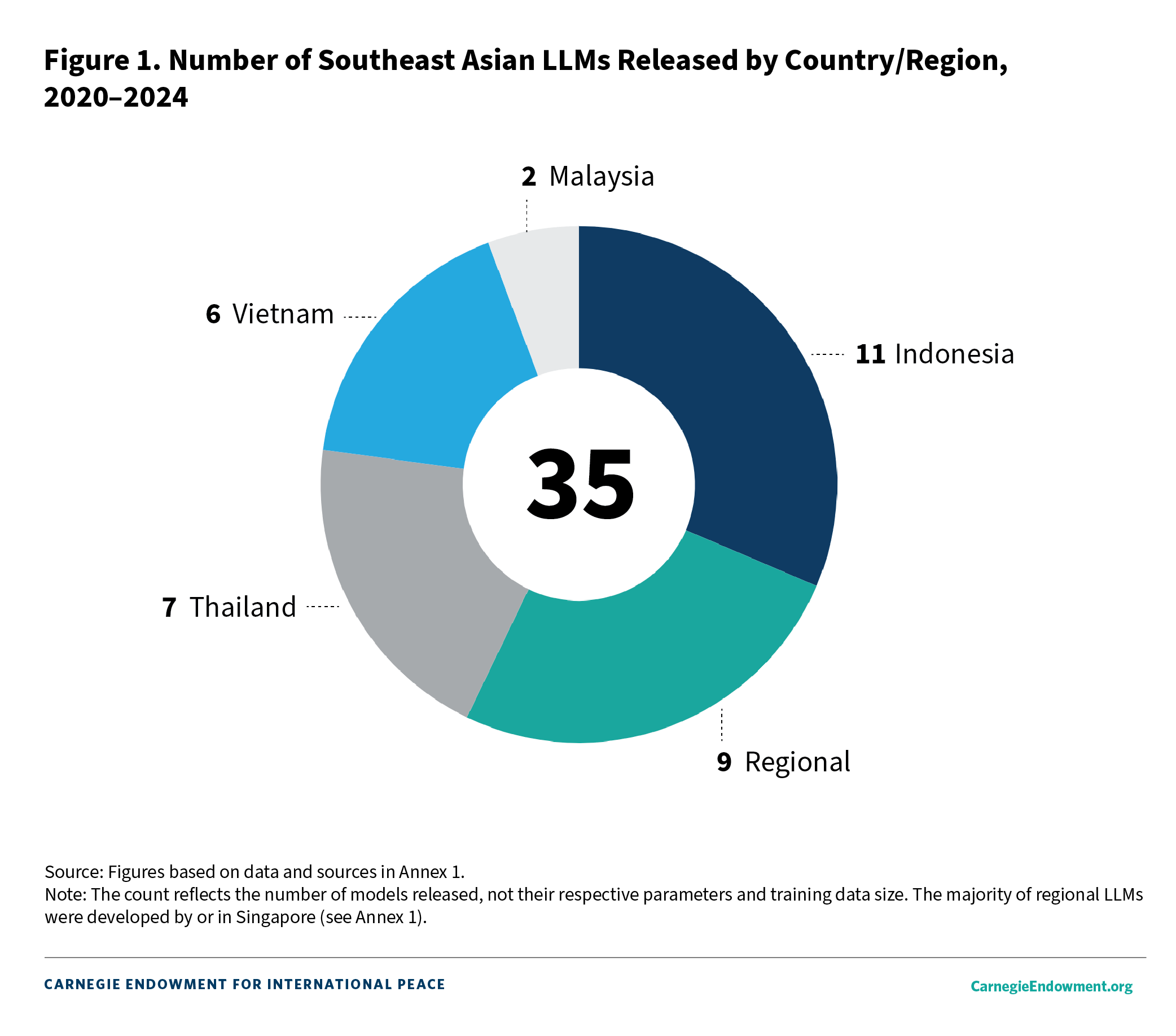
In 2024, the number of regional LLMs released and updated jumped more than threefold from 2023 and sevenfold from the previous year, an indication of not only growing interest but relative ease and skill in developing these initiatives (see figure 2). Part of this growth has been facilitated by the availability of open-source foundational models, which themselves have benefited from data diversity, computational advancement, and algorithmic innovation.22 But, as will be seen below, there has also clearly been interest in Southeast Asian countries at the government, research, and industry levels to accelerate the development of AI, in general, and localized LLMs, in particular.
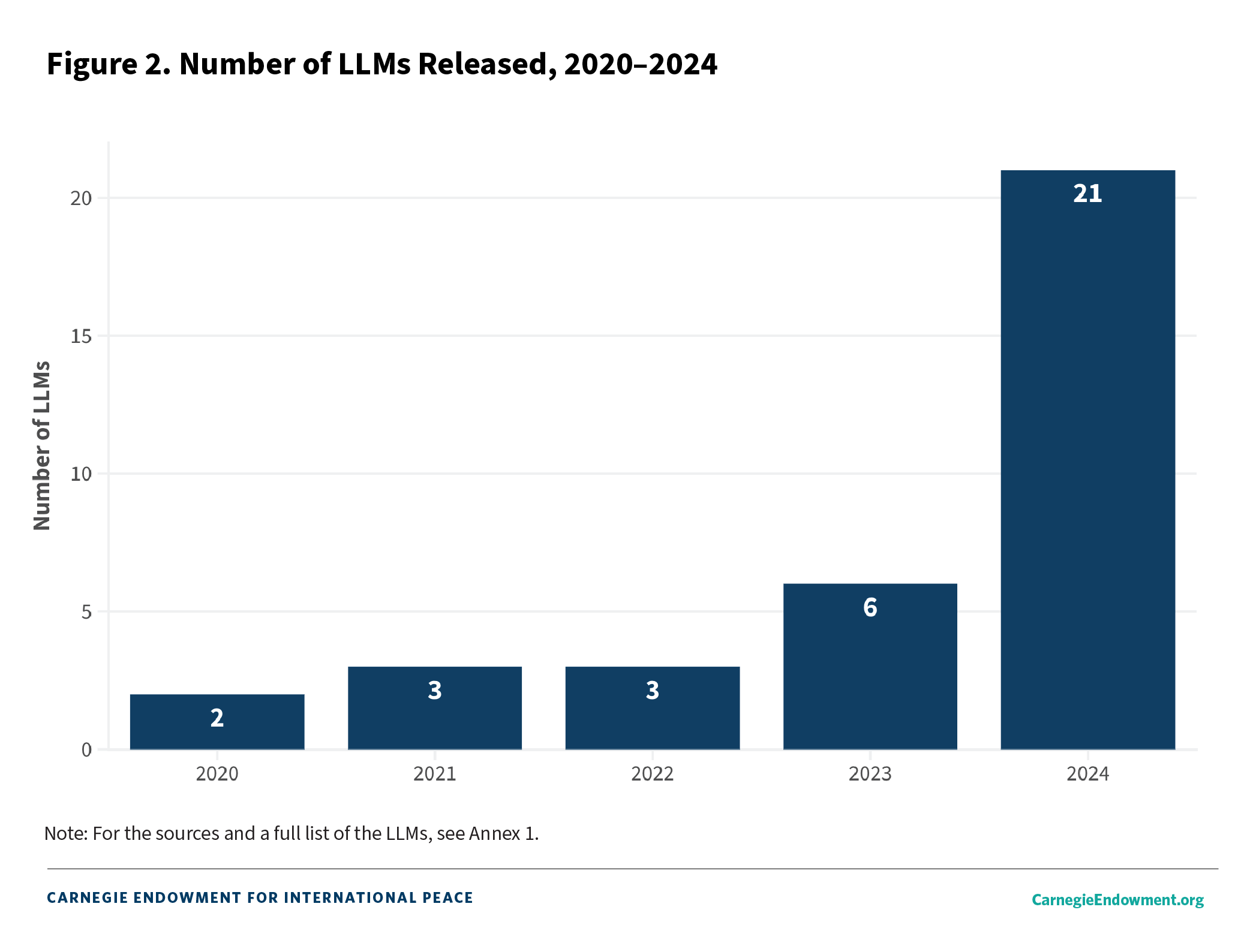
External Models: Lever or Dependence?
When creating an LLM, developers face a choice: pretrain a model from scratch, train a model from scratch, or fine-tune an existing base model. At its simplest, pretraining from scratch teaches a model everything about language from zero. Training from scratch teaches a model one specific skill from zero. These methods give developers greater control over the model’s outputs. Alternatively, developers can fine-tune an open-source model. This involves taking a pretrained model that already knows a lot and giving it focused training for a specific skill or task using a smaller dataset.23
Southeast Asia’s developers have mostly relied on the fine-tuning route, creating or gathering local language datasets to fine-tune advanced, commercial, English-based models (see table 2). This approach is largely driven by cost and convenience calculations given how computationally expensive and data-heavy it is to pretrain models from scratch. Big Tech players have a substantial comparative advantage in this regard. For example, Google’s BERT (Bidirectional Encoder Representations From Transformers), one of the original LLM architectures released in late 2018, already had a parameter size of 110 million (M) for its Base model and 340M for its Large model.24 Parameters refer to variables that configure how a model processes input and produces output. In 2023, the smallest LLaMA (Large Language Model Meta AI) model released by Meta had 7 billion (B) parameters.25
Meta positions LLaMA as an open-source foundational model to be fine-tuned and localized. On one level, openness mitigates the power asymmetries between a handful of mega, well-resourced corporations and smaller, more rudimentary LLM initiatives in other parts of the world. But the veneer of openness can actually perpetuate power imbalances, market centralization, and corporate capture through hidden proprietary practices. For example, whether a model merely offers an application programming interface (API) or full accessibility to its source code makes a difference between a fully open model or one that is superficially open.26 Although Meta’s claim of “publicly available sources” for LLaMA’s pretraining data may convey a sense of transparency, its training dataset is not readily accessible.27
Researchers assessing the openness of different systems have offered a color-coded, multidimensional set of criteria based on, among other things, the availability of source code, data, and weights; scientific documentation on licensing, code, and architecture; as well as a system’s access methods (through package agreements or APIs). Many of the larger base models from big corporations seem to be plagued by issues of undocumented, web-scraped data; little information on the reinforcement learning from human feedback process; and a lack of peer-reviewed credentials. 28
The solution to modifying Western-biased training data along with these models’ embedded logic and cultural context is not always collecting more data since more language data also means more bias. As others have argued, the trade-off of adapting and fine-tuning a local model from much larger models simply shifts homegrown development of more accurate local models to a dependency on established players, at least in the early stages.29
Training From Scratch: Southeast Asia’s Homegrown Foundation Models
In this regard, several regional exceptions stand out in pretraining mono- and multilingual models from scratch: AI Singapore’s SEA-LION, VinAI’s PhoGPT, and Mesolitica’s MaLLaM. The first version of AI Singapore’s SEA-LION was pretrained from scratch using a proprietary tokenizer customized for Southeast Asian languages. In the NLP context, a tokenizer converts a word or stream of text into units of numerical data since models can only process numbers.30 A token is the smallest unit of textual data that a model can process. In the case of SEA-LION, one trillion tokens were used, amounting to 5 terabytes on disk. Although most of SEA-LION’s pretraining data came from the internet, it had to be preprocessed and adjusted for a better reflection of the region’s distribution of languages.
AI Singapore stated that SEA-LION’s training data comprised 13 percent Southeast Asian–language content, 64 percent English-language content, and the remainder Chinese-language content and code.31 Though still trained primarily on English sources, this represents far greater use of Southeast Asian–language training data compared to LLaMA 2’s less than 0.5 percent. Notably, one of the key reasons AI Singapore chose to pretrain SEA-LION from scratch was to ensure that only noncopyrighted data sources were used. Fine-tuning a large, existing model with no disclosure of data sources would risk serious copyright complications down the road.32 The ongoing lawsuits by U.S. and Canadian news outlets against OpenAI for copyright infringement are cautionary tales of data scraping for local LLMs in Southeast Asia.33
PhoGPT, a Vietnamese language model released in November 2023 with 3.7B parameters, was pretrained from scratch on a Vietnamese corpus of 102B tokens. This base model was then fine-tuned on a dataset of instructional prompts and responses as well as nearly 300,000 conversations to launch a chat variant, PhoGPT-4B-Chat.34
MaLLaM, a family of Malay language models of 1.1B, 3B, and 5B parameters, released in January 2024, relied on a dataset encompassing 90 billion tokens sourced from a range of Malaysian contexts. This was done to minimize, if not completely remove, “English-centric biases pervasive in existing language models.”35 Resource-wise, MaLLaM benefited from the efforts of volunteers who built the initial dataset to pretrain the model from scratch, as well as from the support of NVIDIA and Microsoft for advanced computational and technological resources.36
SEA-LION, PhoGPT, and MaLLaM remain anomalies as several of a small set of models in Southeast Asia pretrained from scratch. Paradoxically, given limitations in both data availability and training resources, the best option for regional innovators seems to be to fine-tune more mature, foundational models even as developers are trying to break free from existing biases in those very models.
Implications of Base Model Diversification
From 2020 to 2022 (pre-ChatGPT), all the models in Southeast Asia relied on U.S. base models. In 2023, there began to be a diversification away from U.S. models, with Southeast Asian developers turning to local initiatives; the French model, Mistral; and international collaborative efforts (see figure 3). In 2024, the number of regional LLMs doubled, and Chinese models (specifically Qwen) made a splash, accounting for a quarter of all new models.
The addition of Qwen to the suite of base models used in Southeast Asia raises queries about source bias in its Chinese- and English-language corpora, just as those questions often surface in relation to Western English-language models. If, as some posit, Qwen is preloaded with politically and culturally filtered perspectives aligned to Chinese government sensitivities due to the model’s corporate parentage, Southeast Asia may end up having more than only Western bias to be mindful of.37 This concern is not unique to Qwen, however. Any Chinese language data in other models would also be susceptible to ideological biases, depending on their sources.
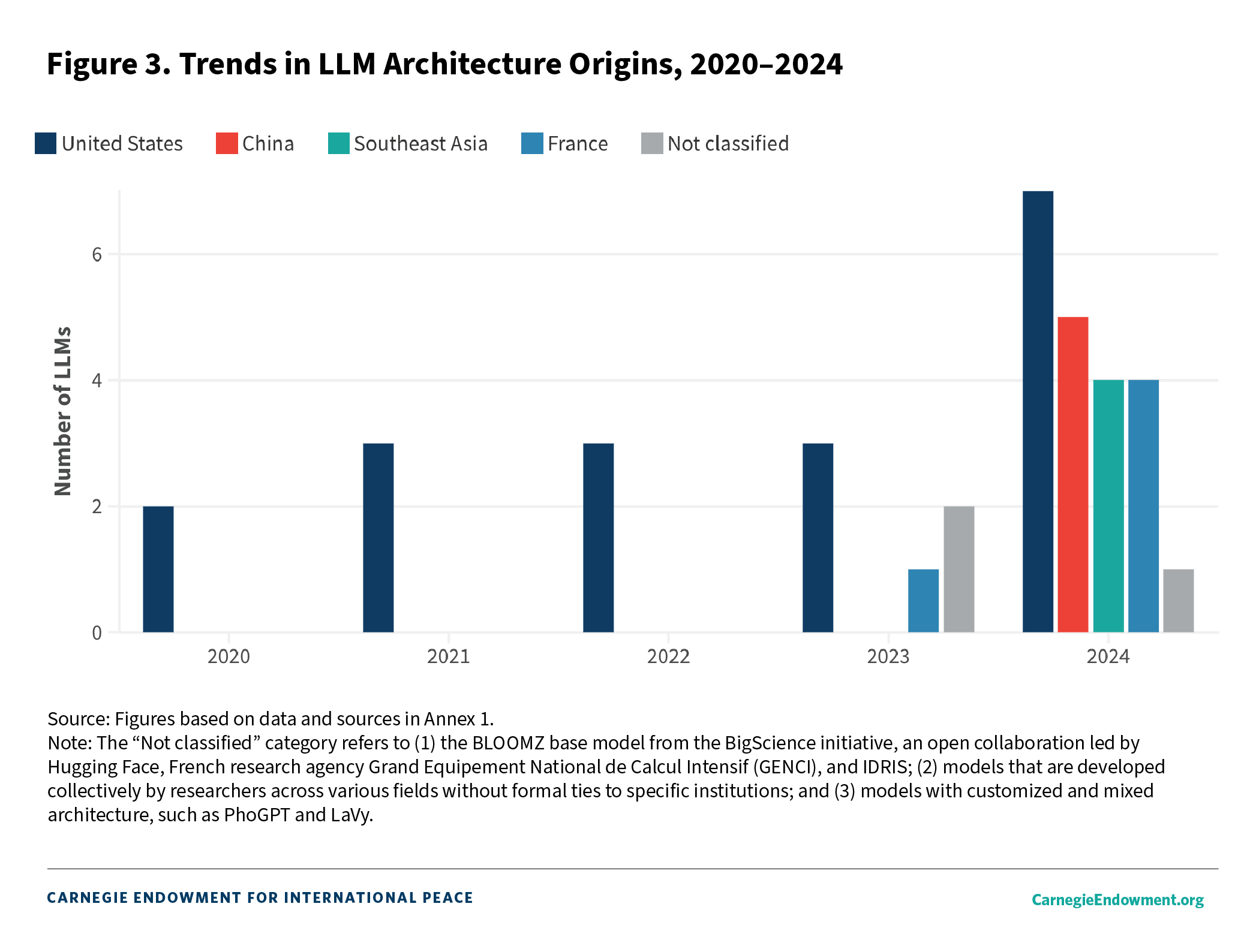
But the shift to diversify foundational models in the region could also point to such models being yet another focus of U.S.-China tech rivalry. Further, with a few exceptions, it is notable that within China and the United States, base model development remains concentrated among a few tech giants able to capitalize on market size to secure exponential economies of scale, thus cementing their dominance. Even Mistral, the French general AI start-up, has entered into a multiyear partnership with Microsoft, giving Mistral access to Microsoft’s supercomputing infrastructure as well as its customers worldwide.38
While this “landscape of leaders” invites a discussion on Southeast Asia’s locus in LLM development in the next ten to twenty years—specifically, whether the region has the appetite or wherewithal to pool resources to eventually build a large, foundational model from scratch for much larger usage beyond the region—an overwhelming number of developers have made it very clear that their goal is not to rival OpenAI or Google in this space.39 Rather, it is to serve unmet or underserved needs in Southeast Asia. As such, most of the regional models developed to date are open source for greater accessibility to the local research community.
It is difficult to assess just how useful or popular regional models have been for developers in Southeast Asia. One metric may be the number of stars the models have received or the number of watchers of these models on GitHub, which is a platform that enables developers to share their code. These numbers indicate the level of interest in the models even though they do not adequately compare regional models against each other. For general reference, as of the time of this writing, LLaMA 3 had 27.5k stars and 229 watchers, while Qwen had 14.7k stars and 110 watchers.40 SEA-LION had 282 stars and 22 watchers, SeaLLM had 152 stars and 14 watchers, and PhoGPT had 760 stars and 21 watchers.41
What is clear is that across the board, developers are as open to collaboration as they are committed to advancing a richer understanding of Southeast Asian languages, perspectives, and culture.42
Contextualizing the Sociopolitical Landscape of Localized LLMs
The development of homegrown LLMs in Southeast Asia has not occurred in a vacuum and is, in part, a product of the sociopolitical realities of the region. Computational linguistics, after all, is a digital derivative of languages used, modulated, and experienced by communities of people. In a region as culturally varied as Southeast Asia—where multiple languages are often effortlessly woven into regular conversation by a single ethnic group of people, where oral rather than written traditions are the norm, where nonverbal cues speak louder than words, where punctuations are sometimes the aberration rather than the norm, and where even the idea of a national language is a recent construct—building LLMs for the region is markedly distinct from building an English-language model. A consideration of the technical design, possibilities, challenges, and trajectories of LLMs in Southeast Asia must, therefore, take into account the region’s broader social, political, and historical complexities.
As prefaced above, language choices and policies have always been highly contested in culturally pluralistic Southeast Asia. Each country also faces distinctly different challenges that set it apart from its neighbors. It is worth briefly surveying these complexities in the five regional countries that have produced localized LLMs, covered in this paper, for a sense of how far these nations have progressed in attempting to project their languages through LLMs, but it is also worth identifying the gaps that remain.
In Malaysia, although the institution of the Malay language in the 1970s as the medium of instruction in all educational institutions was meant to anchor the country’s various ethnic groups to a common form of communication, how the language is even referred to in Malay itself—Bahasa Melayu or Bahasa Malaysia—has been the subject of debate.
The literal translation of Bahasa Melayu is “Malay language.” Article 152(1) of the Malaysian federal constitution, the highest law of the land, specifically refers to Bahasa Melayu as the national language.43 However, in a racially polarized society, a reading of the term as “language of the Malays” can have the unfortunate connotation of underscoring the primacy of the Malay identity and culture over others. Linguists have argued that the literal translation of Bahasa Malaysia as “Malaysian language” does not make sense, because the language is not an aggregation of the languages of the various ethnic groups in the country.44 However, the term conveys to society an intention of unity rather than division and carries hopeful reassurance in the shadows of Malaysia’s traumatic racial riot in 1969, particularly to Malaysia’s minority communities, which make up about 40 percent of the total population.45 Today, Bahasa Melayu retains official acceptance, but the term Bahasa Melayu is still used casually by Malaysians who favor its spirit of conciliation.
By contrast, the choice of Bahasa Indonesia as independent Indonesia’s national language was premised on egalitarian considerations. Bahasa Indonesia itself is a form of Malay that became common vernacular through maritime trade routes between the thirteenth and sixteenth centuries in what is now Southeast Asia. Indonesian nationalists chose it as the country’s language of unity because it was not tied to any colonial power, did not favor the largest ethnic groups in the country, and was not structurally hierarchical like Javanese, for example. The Indonesian language was, therefore, symbolic of the independence movement’s desires for equality and democracy in the fledgling postcolonial country.
And yet, Bahasa Indonesia is not the primary language of socialization for most Indonesians. It has been internally criticized by some as too simple or rigid since it is used in more formal settings. Some associate it with homogeneity and a politically sensitive period given that the language was heavily promoted during former president Suharto’s three-decade-long, heavy-handed rule beginning in the mid-1960s.46 Many Indonesians also have “complex linguistic repertoires” and can dynamically switch between languages in social conversations.47
This code-mixing or code-switching is characteristic of Singlish, a variety of colloquial English in Singapore that intermixes words, grammar, and accents from other local languages. For decades, the use of Singlish has sparked “very open and public disagreements among Singaporeans as to its legitimacy and desirability.”48 At the turn of the millennium, the Singaporean government spearheaded the Speak Good English Movement, aiming initially to minimize if not eradicate Singlish because the government saw it as a threat to the country’s image, economic advancement, and ability to function as a global bridge.49 The government moderated this stance more than a decade on, and today, Singlish is generally celebrated and marketed as uniquely, authentically Singaporean since it transcends class relations.50
In Vietnam, although more than 100 different languages are spoken by some fifty ethnic groups, Vietnamese is the most widely spoken language today even if regional variances can vastly fluctuate. The fact that there is a common language at all is a sharp contrast to the country’s early history when Vietnam’s repeated clashes with various occupying powers meant that it was precluded from developing a national language for the longest time.51
The partition of Vietnam into north and south at the end of the first Indochina war in 1954 led to a split in language policies in the two parts of the country. In the north, Vietnamese became the sole official language at all levels. Chinese, English, French, and Russian were taught as foreign languages, though a natural hierarchy of preferences took shape according to North Vietnam’s political alliances. In the south, French persisted until 1968 when the government instituted Vietnamese as the only language of instruction. English then gradually took hold due to U.S. involvement as well the impact of the British Council and the Colombo Plan, an inter-governmental organization advocating self- and mutual help to “enhance human capital development and south-south cooperation.”52
The 1975 reunification of Vietnam meant the extension of the north’s language policy, including the teaching of Russian, to the whole country. English (and French) subsided in importance, particularly in the south, amid concerns of political recriminations. But Vietnam’s đổi mới (renew or innovate) reforms in the mid-1980s reanimated the importance of English, and by 2005, English made up 99 percent of the foreign languages learned in Vietnamese junior secondary schools.53
Tensions between a country’s national language and English as the lingua franca of education, trade, and commerce overlook the numerous local or indigenous languages that tend to be marginalized in the competition for policy focus, resources, and capacity. Governments may even see the promotion of these other languages as “inimical to the project of promoting national unity and a nation state,” particularly if serious communal fissures already exist.54
Thailand, despite appearances of homogeneity, is home to more than seventy indigenous languages. While the country has been largely successful at inculcating a strong sense of Thai identity among various ethnolinguistic communities that settled from neighboring countries over different periods of time, long-standing clashes between Thailand’s capital Bangkok in the north and Thailand’s restive south over assimilation policies led the government to view the teaching and learning of Pattani-Malay with some suspicion at one time.55
It is this backdrop of the unsettled nation-building project in Southeast Asia that makes the budding development of localized LLMs all the more striking. While the world around shrinks through technology, the region’s stakeholders in government and the technical community are highly cognizant of the importance of holding firm to local perspectives and cultures. This is true even if local viewpoints and values have not yet fully cohered as countries continue to grapple with constructing a national identity.
Opportunities: Representation, Agency, and Educational Advancement
The refrain from regional developers about elevating regional languages, ideas, and social attitudes while redressing the English language and Western bias in presently dominant LLMs speaks volumes about just how far many Southeast Asian nations have come in self-awareness and confidence within barely three generations of attaining independence.56
Mitigating tensions between and among languages spoken in regional states is a delicate balance of reconciling the past and engineering the future. It is a question of how to unite a people while preserving diversity, all while ensuring an adequate mastery of English as the lingua franca of trade and economic development.
Remedying English or Western bias in language models, therefore, does not equate to diminishing the importance of English or shunning engagement with Western standards. Quite the contrary, in Singapore, which has incubated the regional models of SeaLLM, SEA-LION, and Sailor, English was very early on embraced as an ethnically “neutral language” and one that would ensure Singapore’s active participation in the global economy.57
Even where the status of English has been fraught because of its colonial baggage, such as in Malaysia or the Philippines, pragmatism or “linguistic instrumentalism” has prevailed. Indeed, it is English, rather than any or many Southeast Asian language(s), that is the sole working language of the Association of Southeast Asian Nations (ASEAN).58
In some cases, the use of English in casual conversation is preferred for its “qualities of directness and neutrality,” which local or indigenous languages are not inherently set up to convey.59 Having this language choice allows Southeast Asians the flexibility of a different cultural lens and embedded world view, as well as access to knowledge that would not otherwise be available with only one language.
Ensuring a preponderance of Southeast Asian cultural values and, correspondingly, a diminution of English and Western dominance in LLMs, therefore, is reclaiming and re-presenting a regional identity that comports with local expectations even though those expectations have themselves been influenced or changed by English or Western exposure. As discussed below, this is not always easily, accurately, or faithfully depicted in LLMs for technical, philological, or other reasons.
Still, this growing self-assurance in Southeast Asia and the emergence of localized LLMs paves the way for the region to assert greater, practical agency in technological development in the longer term. With the term “agency” on the rise in Southeast Asian policy circles60—depicting the region’s active capacity to influence or renegotiate its terms of strategic engagement with much larger powers for its own interests—locally developed LLMs are a concrete step toward advancing this autonomy in a meaningful way for the region’s population.
Even if Western LLMs continue to dominate the AI space, having a constituency of researchers, engineers, and policymakers with hands-on experience designing and developing models suited for their own national or regional contexts would place Southeast Asian stakeholders in a more informed position to negotiate with, push back on, or make specific demands of Western providers. The creation of region-wide LLMs such as SEA-LION, SeaLLM, and Sailor, as well as organic local models, is a step toward more proactively shaping engagement with much larger and powerful foreign models that could involve tailoring technical or regulatory demands for local compliance. Many of these models continue to benefit from a collaborative network of NLP researchers from the region continually exchanging and refining information. SEA-LION, for example, counts a number of governmental, private sector, and other organizations in the ASEAN region as its partners, as well as established, multinational players such as Google, Sony, Amazon Web Services, and Alibaba. Seeding this habit of cooperation not only enhances collective, technical knowledge in the region, but also builds long-term trust and confidence among ASEAN researchers in an increasingly important field.
Further, since technology is a strategic tool, cultivating exposure to LLM development, fine-tuning skills that are grounded in local expertise, and asserting agency in the AI sphere would afford Southeast Asia greater critical maneuverability in the future were it to find itself in the middle of difficult value chain decisions triggered by geopolitical contestation. Singapore’s vision, as articulated by its education minister, Chan Chun Sing, is for the country to be a “trusted and neutral place where people can bring the best of technology together to collaborate and not just to compete.”61
Countries that have invested resources in developing localized LLMs also stand to benefit reputationally from the success of these models. Singapore, in particular, which has already positioned itself as a regional tech leader, invested 70 million Singapore dollars (about 50 million U.S. dollars) in December 2023 to boost its research and engineering capabilities in multimodal LLMs for the next two years. SEA-LION, a product of AI Singapore, which is itself a “multi-party effort between various economic agencies and academia” is but a first step in a series of continuing efforts to build more sophisticated models in the coming years.62 All this is a part of the country’s National AI Strategy 2.0 to transform into a global AI leader by 2030, particularly in the development and deployment of scalable solutions for citizens and businesses.63 AI Singapore’s senior director of AI products, Leslie Teo, has been emphatic that by working together with different, even geopolitically competitive players, Singaporeans get an “opportunity to play at the global level.”64 Alibaba DAMO Academy, which developed SeaLLM, also has a presence in Singapore. The country’s pitch for these types of innovations is that its small size can be an advantage for AI collaborators as systems can be tested and deployed faster than in larger countries.
Beyond Business: Localized LLMs for Educational Advancement
The commercial case for localized models in Southeast Asia is easy, if not obvious. The region comprises nearly 700 million people, and the ten member states of the ASEAN make up a combined market size of nearly $4 trillion.65 This figure places the region as the fifth-largest economy in the world behind the United States, China, Japan, and Germany.66 There is also significant investor interest and confidence in the AI growth potential of Southeast Asia, especially in Singapore, as seen in figure 4.
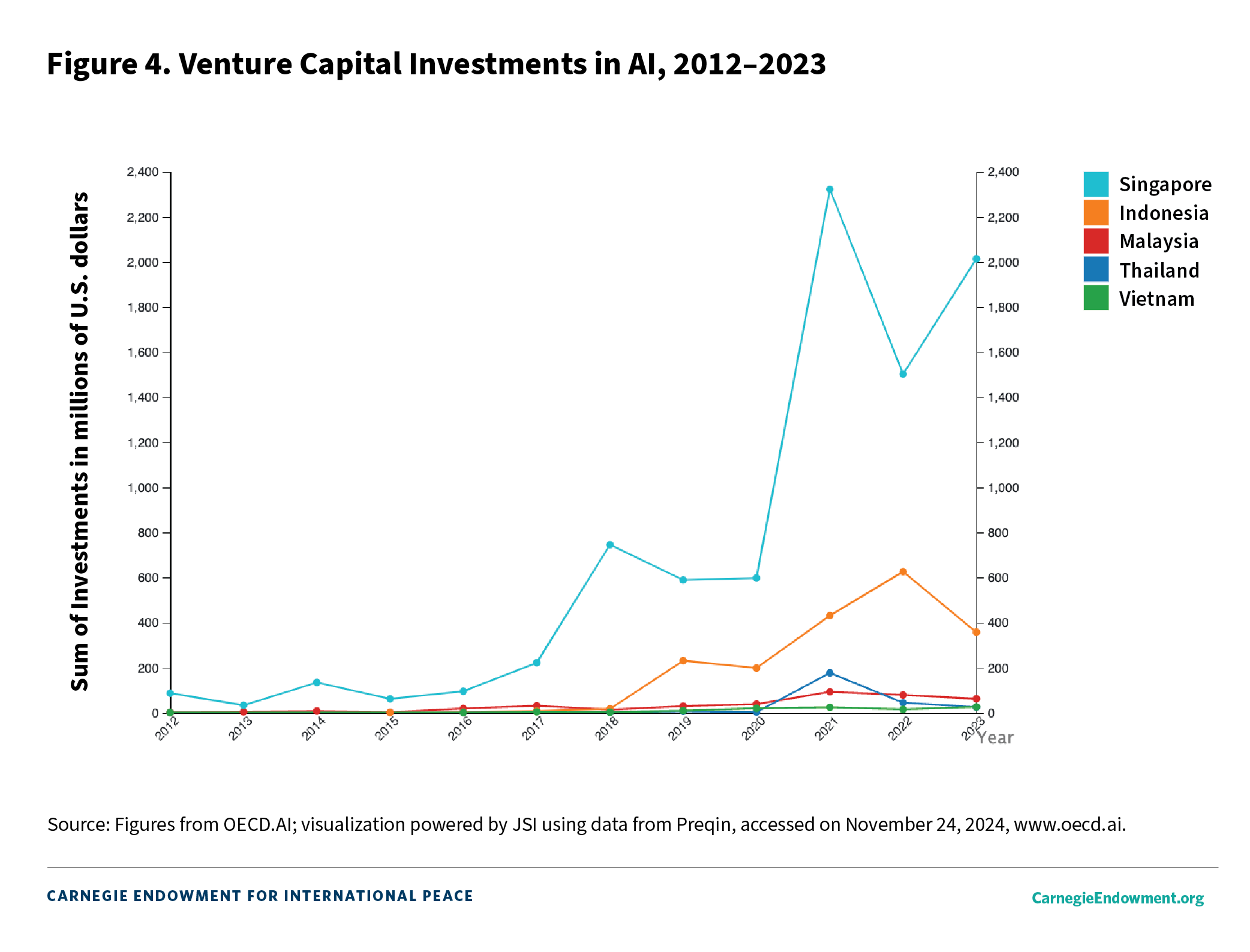
Ensuring the availability of localized LLMs tailored to regional nuances makes sound business sense. After all, ASEAN countries are collectively and individually embarking on ambitious data-driven transformation plans, the region’s digital economy profits have more than doubled since 2022, and consumer interest in AI solutions is rising.67
Already, SEA-LION is available for businesses to customize and deploy for chatbot services, coding assistance, and meeting summarization.68 One of the more valuable ways in which these models could be optimized, however, would be in reducing educational barriers to entry.
Studies show that access to instruction in a language students understand is vital for cognitive development and lifelong success.69 Thailand’s National Language Policy, for example, recognizes the value of mother tongue instruction as foundational for learning other languages and subjects. The country’s National Strategic Plan 2019–2039 also supports languages other than Thai for mainstream education.70
Yet, by one estimate, approximately 40 percent of the world’s population lacks access to education in a language they speak or understand.71 International and regional learning assessments also confirm an adverse impact on test scores when home or mother tongue and school languages differ.72 Much of this inequality occurs where linguistic diversity is the greatest, and poverty and gender can exacerbate educational difficulties.
Although Southeast Asia has advanced pluralistic language-in-education policies in recent decades, the relationship between English, designated official languages, and what educationists term nondominant languages (or other local languages) has been a complex—sometimes tenuous—journey.
For example, while minority languages in Vietnam are recognized in official policy, the teaching and learning of Vietnamese have been strongly privileged as a practical matter to facilitate intercommunal communication. One of the unintended effects of this prioritization has been the relegation of mother tongue languages for minority learners, including those from the Hoa, Muong, and Tay ethnic groups and consequently, educational disadvantages leading to their high dropout and failure rates in school.73
The Malaysian experience also clearly underscores these language tensions. For policymakers, balancing communal pressures from different ethnic constituencies, dignifying Malay, and strengthening English as the language of trade and scientific advancement have resulted in several rounds of reversal of the country’s language education policy since the 1970s when the Malay language replaced English as the medium of instruction in all educational institutions.74
A review by the United Nations Educational, Scientific and Cultural Organization of education plans in forty countries revealed that less than half recognized the importance of teaching children in their home language.75 Localized LLMs could therefore be deployed as a teaching aid in similar situations, especially in the early years of primary-level education. Tailored models could also be used to support the teaching and learning of specialized subjects such as mathematics and science. In this regard, the development of models in Sundanese for an Indonesian setting, for example, could buttress conventional schooling by ensuring learners’ needs are met at a foundational level before children transition to other languages such as Indonesian, English, or Chinese.
Additionally, local content and examples could be used for greater resonance among the intended audience, thus mitigating the limitations of learners’ (and teachers’) grasp of English. In fact, in the early 2000s, Indonesia’s national curricula required local languages such as Sundanese and Javanese to be taught as core or “local content” subjects, supplemented by the development of accompanying teaching and assessment material for effective implementation.76 While this would hardly narrow the gap for the hundreds of other local languages that remain marginal in Indonesia, localized LLMs in a few of the more widely spoken vernaculars could be an important didactic tool for a few million Indonesians at a crucial learning age.
Technical and Substantive Challenges With Localized LLMs
Technical
A large part of the deficiency in advanced, commercial LLMs when it comes to non-English languages is due to the lack of voluminous, high-quality data, even if, like Indonesian, the language is spoken by nearly 200 million people. The underrepresentation of a rich corpus of local data sources, including works of literature in regional languages, could lead not only to a flattening of perspectives on history, heritage, and communication styles but also “the younger generation becoming increasingly disconnected from their roots and . . . a homogenization of thought and expression, with Western perspectives dominating the AI-generated content consumed.”77
Linguistically, the paucity of high-quality, high-quantity data is attributed to lexical, regional, and orthographical variances, even within the same language, as well as unique syntactic or semantic characteristics, among other factors.78 Additionally, social or systemic factors complicate the collection of high-quality data at scale. These include languages being understudied simply because they are not formally taught. For most of these languages, no established standard exists across speakers and there is a scantness in consistently written resources. This informality is compounded by communities intermixing languages in colloquial conversation. In Singapore, Singlish is the country’s most common tongue in everyday conversation. Similarly, Malaysian English, jokingly called Manglish as a reference to mangled English, often comprises words or phrases stitched together in one sentence from Malay, Hokkien, Cantonese, Tamil, and other local languages.
Relatedly, many Southeast Asian—indeed, Asian—languages operate in high-context cultures where nonverbal cues such as facial expressions and body language are as important if not sometimes more important than spoken communication.
These challenges are replicated in efforts by the region’s technical community to develop indigenized LLMs. They are also heightened by other complexities as researchers seek to meaningfully preserve and elevate local languages in these models. In Indonesia, for example, hundreds of languages are at risk of disappearing, largely and indirectly because they are being subsumed or displaced by Bahasa Indonesia (Indonesian) as the national language. Of the country’s 700-plus languages, 440 are considered endangered and twelve as extinct, by one assessment.79
This presents a dilemma because while the choice of Bahasa Indonesia as a standard, unifying language across the archipelagic nation’s approximately 13,000 islands came to be hailed as “straightforward and successful”80—even before but especially after independence—there is now some concern about the loss of language diversity in Indonesia. What this translates into, when building localized LLMs, is not only a data deficit but also a thinness of context to sufficiently represent languages falling into disuse.
As NLP researchers have pointed out, this can lead to models providing unsafe responses, hallucinating (when a model generates output that is false and/or nonsensical due to a range of factors including incorrect assumptions and biased or insufficient data), or being vulnerable to jailbreaks (where safety features of language models are deliberately circumvented by exploiting model biases or other security measures).81
It has also resulted in inefficiency and higher costs during the inference process of fine-tuning models when tokenization happens; that is, when textual data are broken into subcomponents called “tokens” for machine analysis.82 It is worth noting that there are different forms of tokenization. For example, text can be broken up into words or characters (particularly, in non-Latin script). A word can also be divided into syllables or morphemes, which are the smallest units of meaning in language. The morphemes for the word, “cats” would be the noun, “cat” and “-s” to denote the plural form.
However, in the NLP context, there is no universal understanding of what a token is; rather, developers define tokens through their design of a model. As such, where commercial models are used to fine-tune models for localization, tokenization costs can vary according to languages because what constitutes a token depends on training data and the language model.
Research shows that tokenizers of popular, advanced models are biased in favor of Latin-scripted languages and over-fragment less represented languages or scripts. More fragments mean more tokens, which in turn raises costs on already expensive tokenizer software for developers in the region (see figure 5). Further, these tokens do not always perform well on less represented languages despite their relatively higher costs.83
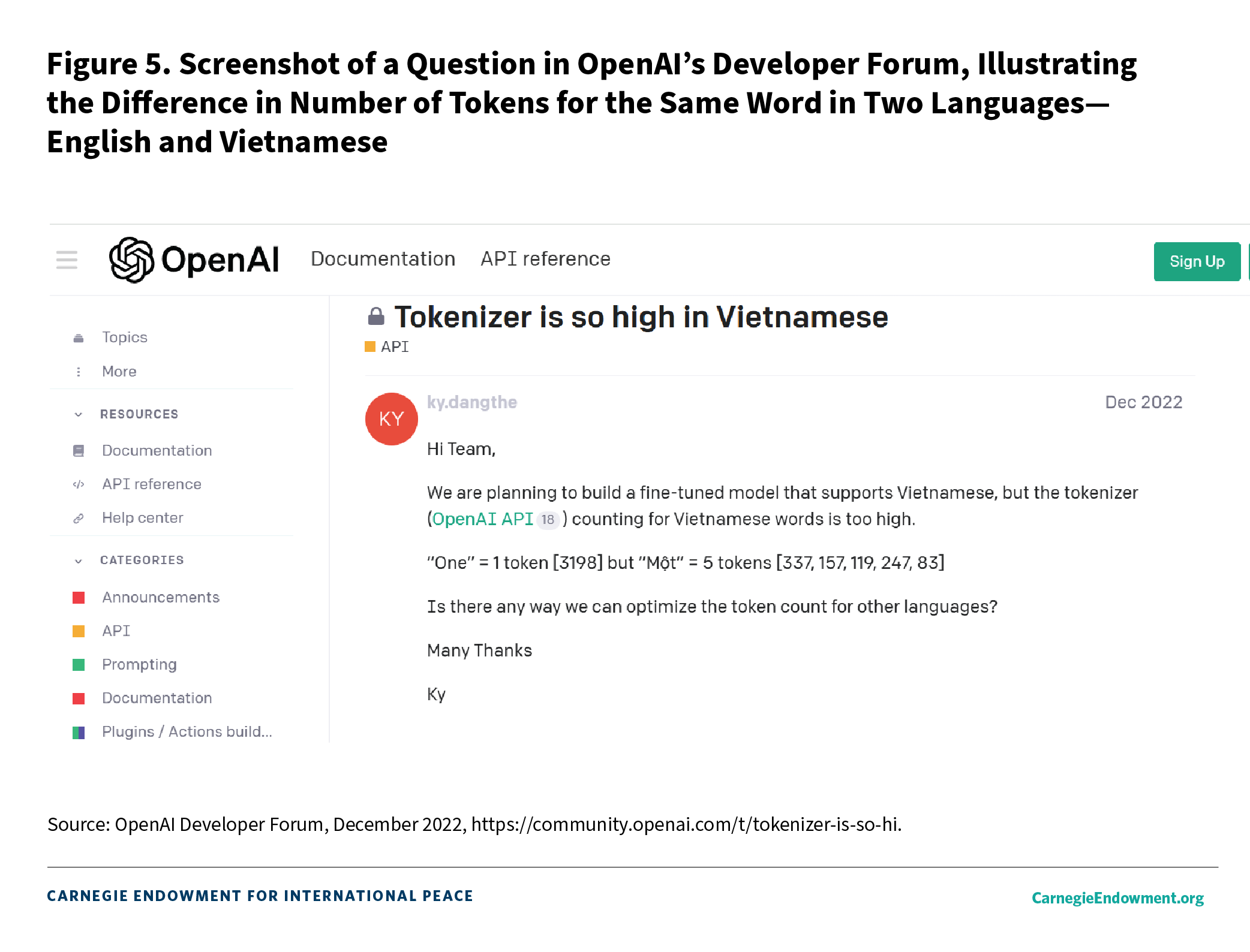
Interestingly, even where colloquial tongues comprising a mix of different languages have been technically challenging for models to break down and reproduce naturally, developers are slowly making some headway. In Singapore, where Singlish has proven tricky for AI voice assistants to process, a health-tech project using Singlish is being trialed with the Singapore Civil Defence Force to help emergency responders transcribe calls. The project’s model has been trained to dynamically transcribe multiple local languages.84 In fact, several other initiatives to train models in Singlish have also already begun. Given the lack of formal documentation in Singlish, training has been done primarily by leveraging larger models to create a synthetic dataset of translated instruction data.85
Data sourcing remains a challenge with these colloquial but commonly spoken forms of English. For example, because Singlish is mostly used in social rather than traditional media or in official speech, there is a risk of anti-establishment bias when Singlish data are obtained.86 This is quite apart from the challenge of filtering only Singlish data from English data since speakers switch between both varieties.87
Substantive
There is a larger, more rudimentary question about what makes Southeast Asian–language models Southeast Asian. This, in turn, impacts the impetus of regional models to preserve local perspectives, knowledge, and cultural values. The obvious answer is that it is the languages of the region that make the models local. But do the data sources of these models inherently reflect endemic knowledge, or do they mirror an interaction with outside perspectives in a way that fundamentally changes local understanding and narratives? Put another way, how much does the underlying data scraped to train home-grown models faithfully mirror local interpretations of even universally assumed notions such as time, space, and nature? Three examples underscore the reality that data are not necessarily knowledge.
The first example relates to an initiative in Indonesia to collect and annotate data, as well as train models in three digitally underrepresented languages: Balinese, Buginese, and Minangkabau. This project relied on community collaboration to develop solutions for local challenges. Because Balinese and Buginese have a rich textual history, written data were not so much a challenge. By writing short texts in their native language, translating, and quality-checking other texts, community members actively contributed to building local datasets. Data annotation also provided remote work opportunities not just for local communities but, equally, for speakers living in urban areas with better access to technology and more stable electricity supply.88
Yet the Balinese sense of history is quite distinct from Western, chronological notions of history. Balinese historical writing establishes and reflects patterns of social and cultural organization so that what might be considered mythical stories, in Western terms, are valid records of history. In Bali, there is no concept equivalent to Western views of fiction—“only degrees of veracity related to the sacredness of form, language, and narrative.”89 If every Balinese text is true, as there would be little point in writing or recording lies or falsehoods, then translating myths or legends for the consumption of users unaccustomed to the Balinese sense of history would require a different way of introducing context (for example, through a more involved and expensive process of tokenization) in LLMs. Reproducing language is one thing, reproducing knowledge and nuance is quite another.
This completely different approach to understanding history has ramifications for other historical records in the region. As historians have noted, those who seek to relate history according to Western models about maritime Southeast Asia, particularly modern-day Indonesia and Malaysia, run into the problem of misinterpretation. Combining information from local texts into a Western chronological narrative lends the risk of misreading historical works as something different than indigenously understood. There is a long tradition of mixing interpretations that dates to eighteenth-century translations of Javanese chronicles, which was continued by British colonial administrator Thomas Stamford Raffles in the nineteenth century.90 In the case of middle or low resource language models, there is a particular hazard of digitally reproducing this misleading pattern and exacerbating the risk of hallucination when models are trained on a mix of English and local data.
The importance of presenting an accurate reflection not only of local languages but also local knowledge bases circles back to the dilemma of training from scratch versus fine-tuning foreign models. If the only feasible way of building local models is to fine-tune other models that will have been predominantly trained on foreign notions of history, then it will be very difficult to undo this flawed understanding that has been baked into the model from the very beginning. If, on the other hand, developers choose to train from scratch, the challenge would be to collect enough high-quality, high-quantity data to train a truly useful model. Data availability would, of course, depend on whether the language in question were a medium or low resource one.
The second example draws from these differences in the conceptualization—and, by extension, expression—of how human beings conduct their everyday life or how governments conduct the business of foreign relations.91 Here, the differences in New Zealand (or Aotearoa) between Māori and Pākehā (or New Zealander of European descent) notions of time and space are illustrative of how ontological logics and their descriptive terms are relative. A word in English can have a markedly different framing in another language. For the Māori, time and space are neither linear nor divisible as in Western thought and practice. Instead, the past, present, and future are collapsed into one, thereby rendering the sequencing and divisibility of time and space as both inappropriate and restrictive.92 There is, therefore, a difference between Māori event-time and Pākehā clock-time, the latter of which was derived from the nineteenth-century British conceptualization of time with values of punctuality, efficiency, and productivity crystallizing at the height of the Industrial Revolution.93 The Balinese order of history, outlined in the first example, parallels the very different, Western understanding of time and space we have come to accept as standard.
In a similar way, the Malay word for “sovereignty” translates into kedaulatan. Yet the etymology of the Malay word is uniquely distinct from how we understand it in English. The root word daulat has its roots in pre-Islamic Hindu-Buddhist origins and signifies a mystical aura of power, authority, and respect that attaches to the ruler rather than a bounded, geographical space. Scholars have argued that in precolonial Malaysia, associated concepts such as reputation, prestige, and status that attached to a sovereign far outweighed concerns of territorially bound rule.94 This understanding has carried over, in part, to modern-day notions of constitutional sovereignty in Malaysia—even arguably contributing to several incidents of domestic political crises.95
Big Tech researchers remind us that as a product of Western epistemology, the goal of NLP research is “to find common aspects or features of languages that can describe large amounts of data in the most general terms, rejecting or ignoring parts of languages that resist easy classification.”96 So, while the design of LLMs in global centers of power, privilege, and prestige may prioritize what linguistic anthropologist Jay Ke-Schutte terms the Angloscene—that is, the distortion of sociocultural views and values by the institutional and aspirational power of English97—Southeast Asian reliance on those base models, as well as a disregard for, or neglect of, the etymology of local lexicon, can ultimately stymie otherwise meaningful attempts at carving AI agency and autonomy for the region.
The third example relates to the more contemporary challenge of collecting and recording data on the climate crisis. While indigenous communities in Southeast Asia may be at the forefront of environmental degradation and climate vulnerability, they do not necessarily possess the lexicon to articulate phenomena such as greenhouse gas emissions or biospheric pollution. This has less to do with the richness of their vocabulary and more to do with the fact that the rapid climate decline has been an externally imposed emergency not of their own doing. The descriptors for this trend, therefore, have not been a natural part of their language and have had to be constructed during the data collection process. This creation of new terms and phrases, in turn, invokes the importation of corollary ideas and explanations from outside rather than within the community. These views risk being re-presented as the community’s own even if they are not, simply because they are described in the local language.
On the one hand, this generation of new lexicon demonstrates the continual evolution of language. On the other, it begs at least two further questions: First, what is it that is really being preserved by training LLMs in local languages if new words must be invented? Second, who is the target audience of this corpus-making? Is it future generations of the community itself or other interested parties? This second question bears special ethical consideration, particularly if the data collected risk being extracted or commercialized for purposes other than the community originally intended, if the workings of AI remain opaque to users, and if the models remain out of reach to most in the community.
African scholars Angella Ndaka and Geci Karuri-Sebina remind us to critically question assumptions of digital inclusion and representation as necessarily positive: “When we volunteer our data and ourselves in the name of digital inclusion, where are we being included? Whose agendas dominate in the technology being developed? And whose technologies are being produced anyway?”98
If what is tabled by the extension of low resource language LLMs amounts to “behavioural ‘nudges’ to increase the consumption of imported products” or ideas, then communities that form part of these new markets have a right to assert agency in the process, including the right to refuse.99 In this regard, the experience of Indigenous communities elsewhere (for example, New Zealand) in preserving and governing data on their language and cultural knowledge could be instructive for local communities in Southeast Asia.
Recommendations
As many people in Southeast Asia’s technical community recognize, constructing models for underrepresented languages is a multifaceted undertaking that requires expertise from different backgrounds. The following recommendations are premised on the argument that LLM development in a region as historically and culturally diverse as Southeast Asia should be carried out comprehensively, involving a range of stakeholders beyond those usually consulted and with a view toward the next few decades rather than just the next few years. As such, Southeast Asian government, industry, academic, and other players could take the following action:
- Increase or expand networks of cross-disciplinary researchers and practitioners. Southeast Asia’s technical community offers a model for partnerships with its open, collaborative approach to researching natural language processing. Expanding this circle to include historians, anthropologists, and other experts from the social sciences and humanities, for example, would surface underconsidered but equally critical dimensions of LLM development relevant to the region and as outlined in this paper’s first and second sections. Similarly, regularizing exchanges with climate specialists or labor activists would round out urgent but oft-ignored deliberations. These include risks to the environment and to labor welfare posed by the entire infrastructural chain of LLMs—from data collection and annotation to the construction and maintenance of data centers that power the training and operation of these language models.100 Substantive consultation between developers and other stakeholders (not focus group market research) is even more important where models are designed or deployed for delicate use cases. A chatbot for an e-commerce site will impact users in a drastically different way than one for therapy among refugee communities, for example.101
- Draw lessons from other regions or communities that are on a comparable trajectory. Building on the above recommendation, researchers working at the intersection of society and technology could look to Africa where regional developers have begun building language models supporting African languages from the ground up by working with local communities. There are already initiatives such as the Africa-Asia AI Policymakers Network that connect government officials in both continents for the purpose of developing responsible and locally relevant AI solutions.102 These initiatives could be replicated at nonofficial levels for two-way exchanges on designing, deploying, and governing local models. Where appropriate, Southeast Asian researchers would also do well to study the relevance of Indigenous community–based projects such as Māori data sovereignty for local application.103
There are also cautionary lessons to heed. Claims of the African Union Commission’s AI strategy having been drafted by “a tech lobbyist from Switzerland” should put Southeast Asian stakeholders on alert as numerous AI-related policies, white papers, and strategies are rolled out in the region without a clear indication of who may be behind them or whose interests they prioritize.104 - Explore the feasibility of multimodal models. Because a large part of communication in Southeast Asia operates in a high-context culture where nontextual cues play a critical role in conveying nuance, regional text-based language models are necessarily limited in scope and effectiveness. Southeast Asian government and technical stakeholders are keenly aware of this limitation, but expanding into multimodal models is resource intensive in terms of costs, energy, and labor, particularly for individual or small teams of researchers.
There are plans to augment existing LLMs in the region. Singapore’s National Multimodal LLM Programme, of which SEA-LION is a part, plans to experiment with techniques to incorporate speech data containing nonverbal cues such as tone and pitch. This will be trialed on nontextual data in standard and colloquial English before expanding to other languages.105 With other models in Southeast Asia relying on SEA-LION as a base model, a multimodal enhancement to SEA-LION could prompt similar improvements elsewhere in the region depending on scale, pricing, and the availability of high-quality data. - Consider or mainstream alternative data governance approaches. Although many countries in the region have data protection laws in place, these are geared toward personal data and are primarily in place to facilitate trade, investment, and commerce rather than to protect the cultural knowledge of any specific community. They are also an inadequate regulatory tool because LLMs typically rely on large, complex datasets that are impossible to disaggregate and language is a community’s cultural asset rather than an individual’s personal one. The notion of protecting community data—particularly, when data on languages of marginalized communities are being collected—should therefore be emphasized. It would also be important at the outset to clarify the ownership of such data and the vesting of decisionmaking authority on such data throughout the collection and annotation processes.
Southeast Asian stakeholders could explore the viability of locally run LLMs, which places or returns the control of models to communities who own the data. Here, indigenous data governance or sovereignty movements in North America and New Zealand offer ready, working examples. Stakeholders could also consider data stewardship arrangements such as data trusts (where trustees exercise data rights of the beneficiaries through a fiduciary relationship) and data cooperatives (where participants jointly pool their data for mutual benefit, including setting the terms of monetizing their data if they choose). - Red-team the purpose and value of LLM optimization. While there is strong forward momentum on LLM localization in Southeast Asia, it is worth reflecting on the limitations of language models and critically asking what the end goal of optimization really is or what the opportunity costs truly are. How many Southeast Asians cannot fluently communicate in the underrepresented languages of their own communities or nations yet expect LLMs to do the same?106 Would resources be better spent on training human rather than computational resources to this end?
Language models are ultimately statistical calculations of word patterns; they achieve success “in tasks that can be approached by manipulating linguistic form.”107 Focusing on leaderboard results can distract from what NLP researchers term “value sensitive design”—that is, designing systems that advance the values of direct and indirect stakeholders from the beginning rather than as a posthoc discovery of risks.108 This will take not only time and transparency but a realignment of research goals with what matters to the people, that technology is supposed to serve a worthwhile goal. In a field where moving fast and breaking things is the mantra of innovation, it can be of greater benefit for AI safety-type organizations in Southeast Asia as well as other stakeholders to rather think deeply and build together. It should be perfectly acceptable—indeed, encouraged—to question the seeming inevitability or necessity of LLMs in the region without the debilitating fear of losing out.
Acknowledgments
The authors benefited tremendously from numerous in-person and virtual consultations with experts from the technical, academic, policy, legal, and other communities in and beyond Southeast Asia. We hope that this paper invites further conversation and study on regional LLMs.
This research was made possible by funding from the Patrick J. McGovern Foundation.
Glossary
Language models are models trained on data to predict the likelihood of patterns or sequences of words based on context. Development in this field evolved from statistical language models to neural language models and more recently, large language models. 109
Large language models (LLMs) are a type of language model trained on extensive datasets and parameters to understand and generate natural language to the point of having emergent abilities that are not present in small models. These abilities include in-context learning, instruction following, and step-by-step reasoning.110
Pretraining is an initial stage of building a large language model. It involves data collection and preparation, as well as training the model on a large corpus of data to create a base model that has language processing capability (see figure 6).111 LLM initiatives in Southeast Asian languages mostly use existing open-source pretrained base models such as LLaMA and Qwen.
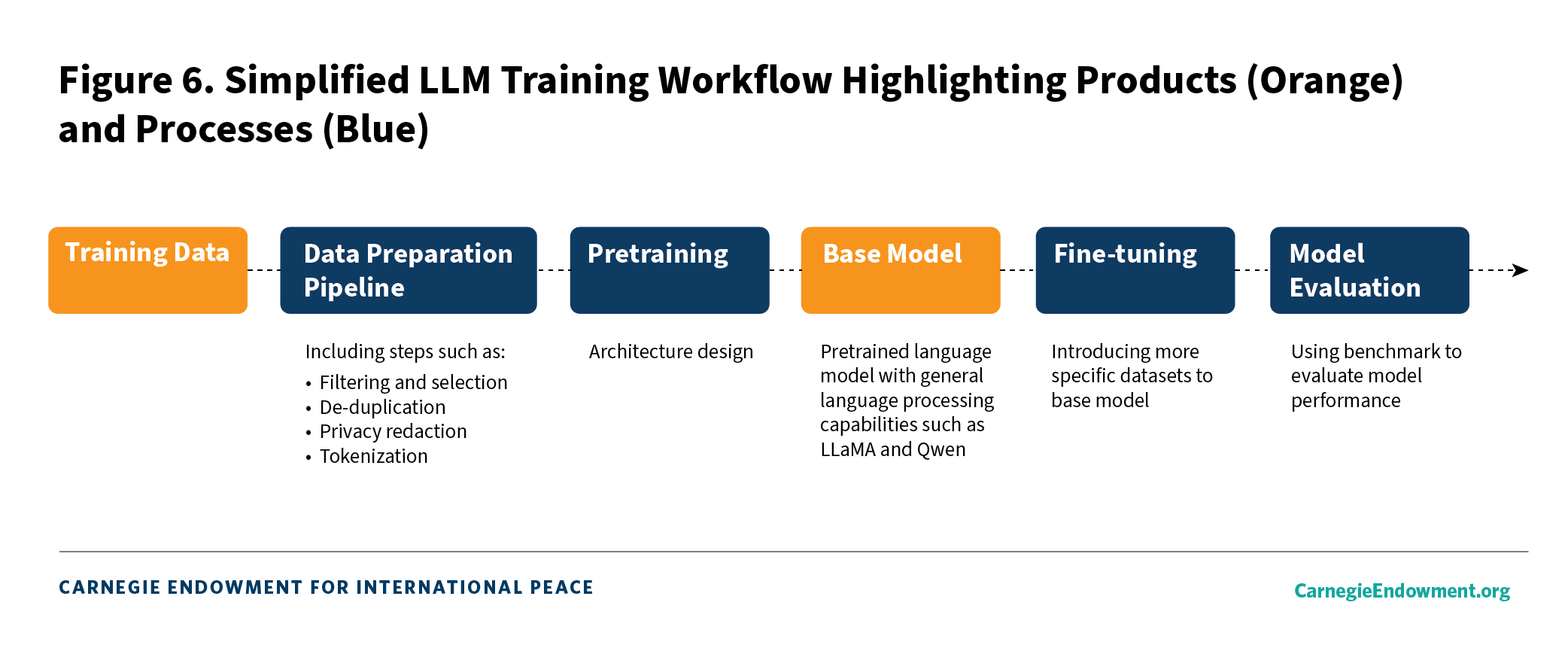
Data preparation pipeline is the process of preparing data for model training. The process involves steps such as filtering and selection, de-duplication, privacy redaction, and tokenization. These steps are to ensure that the data used in training are representative, free of harmful content, and can be computed by the machine.112
Tokenization is the process of segmenting and processing raw text into machine-readable input. There are different methods of tokenization, and each leads to different segmentation and token results.113
Architecture is the specific design and organization of a model’s components (for example, layers, attention mechanisms, and activation functions) that determine how the model processes data and learns from it. The different architecture designs are linked to the performance, training time, memory, and stability of the model.114 Architecture design is one of the steps in training from scratch. Southeast Asian LLM initiatives that are trained from scratch also use existing architecture design (for example, MaLLaM uses the MPT architecture).
Parameter is a tunable value or variable within the model that influences how the model processes input and output. While more parameters can increase a model’s capacity to represent complex patterns, this does not directly translate to better performance. Additionally, larger parameter counts incur high computational costs, requiring significant memory and properly scaled datasets.115
Fine-tuning is a process of introducing more specific datasets to the base model for greater adaptation to a specific goal. Adaptation can take the form of specific languages, domains, alignment, or instructions.116 Most LLM initiatives in Southeast Asia fine-tune existing base models with data of local language or context.
Benchmark is a standard dataset or set of tasks used to evaluate the performance of a model, allowing model assessment and a comparison across different models.117 Annex 1 below provides examples of benchmark initiatives in Southeast Asia for multi- and monolingual models focused on regional languages.
Application Programming Interface (API) is “a set of rules or protocols that enables software applications to communicate with each other to exchange data, features and functionality.”118
Notes
1Longxu Dou, Qian Liu, Guangtao Zeng, Jia Guo, Jiahui Zhou, et al., “Sailor: Open Language Models for South-East Asia,” arXiv, April 4, 2024, https://doi.org/10.48550/arXiv.2404.03608.
2“SeaLLMs—Language Models for Southeast Asian Languages,” Hugging Face, accessed November 4, 2024, https://huggingface.co/SeaLLMs.
3“Why SEA-LION,” SEA-LION.AI.
4OpenAI, Josh Achiam, Steven Adler, Sandhini Agarwal, Lama Ahmad, et al., “GPT-4 Technical Report,” arXiv, March 4, 2024, https://doi.org/10.48550/arXiv.2303.08774.
5Anisa Menur, “How SEA-LION Aims to Bridge the Cultural Gap Existing in Popular AI Tools,” e27, June 20, 2024, https://e27.co/how-sea-lion-aims-to-bridge-the-cultural-gap-existing-in-popular-ai-tools-20240131/; Paresh Dave, “ChatGPT Is Cutting Non-English Languages Out of the AI Revolution,” Wired, May 31, 2023, https://www.wired.com/story/chatgpt-non-english-languages-ai-revolution/; and “Is ChatGPT Biased?,” OpenAI, accessed November 7, 2024, https://help.openai.com/en/articles/8313359-is-chatgpt-biased.
6Kimmo Kosonen, “Language Policy and Education in Southeast Asia,” in Language Policy and Political Issues in Education, ed. Teresa L. McCarty and Stephen May (Cham: Springer International Publishing, 2017), 477–90, https://doi.org/10.1007/978-3-319-02344-1_35.
7David M. Eberhard, Gary F. Simons, and Charles D. Fennig, Ethnologue: Languages of the World, 24th Edition (Dallas, Texas: SIL International, 2021).
8Kosonen, “Language Policy and Education in Southeast Asia.”
9LLMs and AI, more generally, also often influence social decisions and attitudes, thus creating an ongoing feedback loop between technology and society. For more on the sociotechnical approach to AI, see, for example, Brian J. Chen and Jacob Metcalf, “Explainer: A Sociotechnical Approach to AI Policy,” Data & Society, May 2024, https://datasociety.net/wp-content/uploads/2024/05/DS_Sociotechnical-Approach_to_AI_Policy.pdf; Fernando Filgueiras, Ricardo Fabrino Mendonça, and Virgílio Almeida, “Governing Artificial Intelligence Through a Sociotechnical Lens,” IEEE Internet Computing 27, no. 5 (2023): 49–52, https://doi.org/10.1109/MIC.2023.3310110; and Aubra Anthony, Lakshmee Sharma, and Elina Noor, “Advancing a More Global Agenda for Trustworthy Artificial Intelligence,” Carnegie Endowment for International Peace, April 30, 2024, https://carnegieendowment.org/research/2024/04/advancing-a-more-global-agenda-for-trustworthy-artificial-intelligence?lang=en.
10Shahidul Alam, “Majority World: Challenging the West’s Rhetoric of Democracy,” Amerasia Journal 34, no. 1 (2008): 87–98, https://doi.org/10.17953/amer.34.1.l3176027k4q614v5.
11Khalil Nooh, “Mesolitica’s Mission to Build a Localised Malaysian AI Model,” BFM, October 2, 2024, https://bfm.my/podcast/enterprise/open-for-business/mesoliticas-mission-to-build-a-localised-malaysian-ai-model; Dhamir Raniah Kiasati Desrul and Ade Romadhony, “Abusive Language Detection on Indonesian Online News Comments,” IEEE Xplore, 2019 International Seminar on Research of Information Technology and Intelligent Systems, December 5–6, 2019, 320–25, https://doi.org/10.1109/ISRITI48646.2019.9034620; Media A. Ayu, Sony Surya Wijaya, and Teddy Mantoro, “An Automatic Lexicon Generation for Indonesian News Sentiment Analysis: A Case on Governor Elections in Indonesia,” Indonesian Journal of Electrical Engineering and Computer Science 16, no. 3 (2019): 1555–61, https://doi.org/10.11591/ijeecs.v16.i3.pp1555-1561; Hadi Syah Putra, Rahmad Mahendra, and Fariz Darari, “BudayaKB: Extraction of Cultural Heritage Entities from Heterogeneous Formats,” Proceedings of the 9th International Conference on Web Intelligence, Mining and Semantics, Association for Computing Machinery, 2019, 1–9, https://doi.org/10.1145/3326467.3326487; and Ika Alfina, Rio Mulia, Mohamad Ivan Fanany, and Yudo Ekanata, “Hate Speech Detection in the Indonesian Language: A Dataset and Preliminary Study,” 2017 International Conference on Advanced Computer Science and Information Systems, 2017, 233–38, https://doi.org/10.1109/ICACSIS.2017.8355039.
12Elina Noor and Mark Bryan Manantan, “Raising Standards: Ethical Artificial Intelligence and Data for Inclusive Development in Southeast Asia,” Asia Society Policy Institute, July 2022, https://asiasociety.org/policy-institute/raising-standards-data-ai-southeast-asia.
13Simon Huang, “Why Singapore’s LLM Isn’t Sweating GPT-4,” Tech in Asia, January 31, 2024, https://www.techinasia.com/singapores-seafocused-llm-isnt-sweating-gpt4.
14Amal Shiyas, “Microsoft Research Project Helps Languages Survive—and Thrive,” Microsoft, January 30, 2023, https://news.microsoft.com/source/asia/features/microsoft-research-project-helps-languages-survive-and-thrive/.
15Jacob Devlin, Ming-Wei Chang, Kenton Lee, and Kristina Toutanova, “BERT: Pre-Training of Deep Bidirectional Transformers for Language Understanding,” arXiv, May 24, 2019, https://arxiv.org/pdf/1810.04805; and Jacob Devlin, “bert/multilingual.md,” GitHub, https://github.com/google-research/bert/blob/master/multilingual.md.
16“How to Change Your Language Setting in ChatGPT,” OpenAI, https://help.openai.com/en/articles/8357869-how-to-change-your-language-setting-in-chatgpt#h_513834920e, accessed December 1, 2024.
17For more general evaluations on M-Bert’s and ChatGPT’s multilingualism, see, for example, Telmo Pires, Eva Schlinger, and Dan Garette, “How Multilingual Is Multilingual BERT?,” Proceedings of the 57th Annual Meeting of the Association for Computational Linguistics, 4996–5001, Florence, Italy, Association for Computational Linguistics, July 2019, https://aclanthology.org/P19-1493.pdf; Viet Dac Lai, Nghia Trung Ngo, Amir Pouran Ben Veyseh, Hieu Man, Franck Dernoncourt, et al., “ChatGPT Beyond English: Towards a Comprehensive Evaluation of Large Language Models in Multilingual Learning,” arXiv, April 12, 2023, https://arxiv.org/pdf/2304.05613; Shijie Wu and Mark Drezde, “Are All Languages Created Equal in Multilingual BERT?,” arXiv, October 1, 2020, https://doi.org/10.48550/arXiv.2005.09093.
18Yejin Bang, Samuel Cahyawijaya, Nayeon Lee, Wenliang Dai, Dan Su, et al., “A Multitask, Multilingual, Multimodal Evaluation of ChatGPT on Reasoning, Hallucination, and Interactivity,” arXiv, November 28, 2023, https://arxiv.org/pdf/2302.04023; and Paresh Dave, “ChatGPT Is Cutting Non-English Languages Out of the AI Revolution,” Wired, May 31, 2023, https://www.wired.com/story/chatgpt-non-english-languages-ai-revolution/.
19Zheng-Xin Yong, Ruochen Zhang, Jessica Zosa Forde, Skyler Wang, Arjun Subramonian, et al., “Prompting Multilingual Large Language Models to Generate Code-Mixed Texts: The Case of South East Asian Languages,” Proceedings of the 6th Workshop on Computational Approaches to Linguistic Code-Switching, December 7, 2023, 43–63, https://aclanthology.org/2023.calcs-1.5.pdf.
20Yiran Zhao, Wenxuan Zhang, Guizhen Chen, Kenji Kawaguchi, and Lidong Bing, “How Do Large Language Models Handle Multilingualism?,” arXiv, November 10, 2024, https://doi.org/10.48550/arXiv.2402.18815.
21Author’s in-person interviews with NLP researchers, Singapore and Indonesia, July 2024.
22Zichong Wang, Zhibo Chu, Thang Viet Doan, Shiwen Ni, Min Yang, et al., “History, Development, and Principles of Large Language Models: An Introductory Survey,” AI and Ethics, October 14, 2024, https://doi.org/10.1007/s43681-024-00583-7.
23Authors’ email and text communication with NLP researchers, December 2024.
24Parameters refer to the number of learnable variables or values available for the model. Britney Muller, “BERT 101—State of the Art NLP Model Explained,” Hugging Face (blog), March 2, 2022, https://huggingface.co/blog/bert-101.
25“Introducing LLaMA: A Foundational, 65-Billion-Parameter Language Model,” Meta, February 24, 2023, https://ai.meta.com/blog/large-language-model-llama-meta-ai/.
26David Gray Widder, Sarah West, and Meredith Whittaker, “Open (For Business): Big Tech, Concentrated Power, and the Political Economy of Open AI,” SSRN Scholarly Paper, August 17, 2023, https://doi.org/10.2139/ssrn.4543807.
27“Introducing Meta Llama 3: The Most Capable Openly Available LLM to Date,” Meta AI, April 18, 2023, https://ai.meta.com/blog/meta-llama-3/.
28Andreas Liesenfeld, Alianda Lopez, and Mark Dingemanse, “Opening Up ChatGPT: Tracking Openness, Transparency, and Accountability in Instruction-Tuned Text Generators,” Proceedings of the 5th International Conference on Conversational User Interfaces, Association for Computing Machinery, 2023, 1–6, https://doi.org/10.1145/3571884.3604316.
29Eryk Salvaggio, “A Meta Analysis,” Substack, Cybernetic Forests (blog), October 3, 2024, https://cyberneticforests.substack.com/p/a-meta-analysis.
30“Tokenizer,” Hugging Face NLP Course, https://huggingface.co/learn/nlp-course/en/chapter2/4, accessed December 23, 2024.
31Huang, “Why Singapore’s LLM Isn’t Sweating GPT-4.”
32Aaron Tan, “Sea-Lion Explained: Southeast Asia’s First Large Language Model,” Computer Weekly, February 5, 2024, https://www.computerweekly.com/feature/Sea-Lion-explained-Southeast-Asias-first-large-language-model.
33Michael M. Grynbaum and Ryan Mac, “The Times Sues OpenAI and Microsoft Over A.I. Use of Copyrighted Work,” New York Times, December 27, 2023, https://www.nytimes.com/2023/12/27/business/media/new-york-times-open-ai-microsoft-lawsuit.html; and David Ljunggren, “Canadian News Companies Challenge OpenAI Over Alleged Copyright Breaches,” Reuters, November 29, 2024, https://www.reuters.com/sustainability/boards-policy-regulation/major-canadian-news-media-companies-launch-legal-action-against-openai-2024-11-29/.
34Nguyen et al., “PhoGPT.”
35Husein Zolkepli, Aisyah Razak, Kamarul Adha, and Ariff Nazhan, “MaLLaM—Malaysia Large Language Model,” arXiv.org, January 26, 2024, https://arxiv.org/abs/2401.14680v2.
36“Mesolitica’s Mission to Build a Localised Malaysian AI Model.”
37Author in-person communication with government and policy interlocutors, United States and Taiwan, July 2024.
38Eric Boyd, “Microsoft and Mistral AI Announce New Partnership to Accelerate AI Innovation and Introduce Mistral Large First on Azure,” Microsoft Azure Blog (blog), February 26, 2024, https://azure.microsoft.com/en-us/blog/microsoft-and-mistral-ai-announce-new-partnership-to-accelerate-ai-innovation-and-introduce-mistral-large-first-on-azure/.
39Huang, “Why Singapore’s LLM Isn’t Sweating GPT-4.”
40“The Official Meta Llama 3 GitHub site,” GitHub, https://github.com/meta-llama/llama3; and “The Official Repo of Qwen (通义千问) Chat & Pretrained Large Language Model Proposed by Alibaba Cloud,” Github, https://github.com/QwenLM/Qwen, accessed December 11, 2024.
41“South-East Asia Large Language Models,” GitHub, https://github.com/aisingapore/sealion; “[ACL 2024 Demo] SeaLLMs—Large Language Models for Southeast Asia,” GitHub, https://github.com/DAMO-NLP-SG/SeaLLMs; and “PhoGPT: Generative Pre-Training for Vietnamese (2023),” GitHub, https://github.com/VinAIResearch/PhoGPT, accessed December 11, 2024.
42Author in-person interviews with NLP researchers, Singapore and Indonesia, July 2024.
43“Undang-undang Malaysia: Perlembagaan Persekutuan,” Laws of Malaysia, Federal Constitution, Perkara 152 (1) or Article 152 (1); and Zarrah Morden, “Report: Language Expert Says Bahasa Malaysia Cannot Replace Bahasa Melayu,” Malay Mail, May 23, 2022, https://www.malaymail.com/news/malaysia/2022/05/23/report-language-expert-says-bahasa-malaysia-cannot-replace-bahasa-melayu/8290.
44Soo Wern Jun, “Bahasa Melayu or Bahasa Malaysia? As Putrajaya Tightens Reins on National Language, Linguistic Experts Argue Why It Should Be the Former,” Malay Mail, February 12, 2024, https://www.malaymail.com/news/malaysia/2024/02/12/bahasa-melayu-or-bahasa-malaysia-as-putrajaya-tightens-reins-on-national-language-linguistic-experts-argue-why-it-should-be-the-former/112951; and David Fettling, “Why No-One Speaks Indonesia’s Language,” BBC, July 4, 2018, https://www.bbc.com/travel/article/20180703-why-no-one-speaks-indonesias-language.
45The Department of Statistics Malaysia lists a total population count of 34,058,800 for the year 2024. Of that figure, 12,854,200 people are ethnically categorized as non-Malay, amounting to 37.7 percent of all citizens. OpenDOSM, “Population Table: Malaysia,” Department of Statistics Malaysia, https://open.dosm.gov.my/data-catalogue/population_malaysia, accessed December 23, 2024.
46Fettling, “Why No-One Speaks Indonesia’s Language.”
47Michelle Kohler, “Language Education Policy in Indonesia—A Struggle for Unity in Diversity,” in The Routledge International Handbook of Language Education Policy in Asia, by Andy Kirkpatrick and Anthony J. Liddicoat, ed. Andy Kirkpatrick and Anthony J. Liddicoat, 1st ed. (Abingdon, Oxon; NY: Routledge, 2019), 286–97, https://doi.org/10.4324/9781315666235.
48Lionel Wee, The Singlish Controversy: Language, Culture and Identity in a Globalizing World, 1st ed. (Cambridge University Press, 2018), https://doi.org/10.1017/9781316855331.
49Joshua Babcock, “‘Tell Me a Singlish Joke’: Making and Breaking Linguistic-National Boundaries Through ChatGPT in Singapore—and Beyond,” Anthropology News, June 18, 2024, https://www.anthropology-news.org/articles/tell-me-a-singlish-joke-making-and-breaking-linguistic-national-boundaries-through-chatgpt-in-singapore-and-beyond/.
50Velda Khoo, “Voicing Singlish From the ‘Middle’: Indexical Hybridities of Class, Race, Language, and Singaporeanness,” Journal of Linguistic Anthropology 33, no. 2 (2023): 202–22, https://doi.org/10.1111/jola.12403.
51Xuan Nhat Chi Mai Nguyen and Van Huy Nguyen, “Language Education Policy in Vietnam,” in The Routledge International Handbook of Language Education Policy in Asia, by Andy Kirkpatrick and Anthony J. Liddicoat, ed. Andy Kirkpatrick and Anthony J. Liddicoat, 1st ed. (Abingdon, Oxon; NY: Routledge, 2019), 185–201, https://doi.org/10.4324/9781315666235.
52Andy Kirkpatrick and Anthony J. Liddicoat, “Language Education Policy and Practice in East and Southeast Asia,” Language Teaching 50, no. 2 (2017): 155–88, https://doi.org/10.1017/S0261444817000027; and “About The Colombo Plan,” The Colombo Plan Secretariat, https://colombo-plan.org/, accessed December 23, 2024.
53Do Huy Thinh, “The Role of English in Vietnam’s Foreign Language Policy: A Brief History,” Worldwide Translations, February 1, 2007, https://worldwide.rs/en/role-english-vietnams-foreign-language-policy-brief-history/.
54Andy Kirkpatrick and Anthony J. Liddicoat, “Language Education Policy in Asia: An Overview,” in The Routledge International Handbook of Language Education Policy in Asia, by Andy Kirkpatrick and Anthony J. Liddicoat, ed. Andy Kirkpatrick and Anthony J. Liddicoat, 1st ed. (Abingdon, Oxon; NY: Routledge, 2019), 3–13, https://doi.org/10.4324/9781315666235.
55Jacob I. Ricks, “Proud to Be Thai: The Puzzling Absence of Ethnicity-Based Political Cleavages in Northeastern Thailand,” Pacific Affairs 92, no. 2 (2019): 257–85, https://doi.org/10.5509/2019922257; and John Draper, “Language Education Policy in Thailand,” in The Routledge International Handbook of Language Education Policy in Asia, by Andy Kirkpatrick and Anthony J. Liddicoat, ed. Andy Kirkpatrick and Anthony J. Liddicoat, 1st ed. (Abingdon, Oxon; NY: Routledge, 2019), 229–42, https://doi.org/10.4324/9781315666235.
56“AI Singapore,” accessed November 29, 2024, https://aisingapore.org/; Sang T. Truong, Duc Q. Nguyen, Toan Nguyen, Dong D. Le, Nhi N. Truong, et al., “Crossing Linguistic Horizons: Finetuning and Comprehensive Evaluation of Vietnamese Large Language Models,” Stanford AI Lab Blog (blog), July 29, 2024, https://ai.stanford.edu/blog/crossing-linguistic-horizon/; and Zolkepli et al., “MaLLaM—Malaysia Large Language Model.”
57Antonio L. Rappa and Lionel Wee, Language Policy and Modernity in Southeast Asia: Malaysia, the Philippines, Singapore, and Thailand (Boston, MA: Springer US, 2006), 5, https://doi.org/10.1007/0-387-32186-1.
58Catherine Young and Tony Igcalinos, “Language-in-Education Policy Development in the Philippines,” in The Routledge International Handbook of Language Education Policy in Asia, by Andy Kirkpatrick Anthony J. Liddicoat, ed. Andy Kirkpatrick and Anthony J. Liddicoat, 1st ed. (Abingdon, Oxon; NY: Routledge, 2019), 170, https://doi.org/10.4324/9781315666235; and Rappa and Wee, Language Policy and Modernity in Southeast Asia, 125–39.
59Lee Su Kim, “Silent Border Crossings, the Unspoken ESL Dilemma,” in Border Crossings: Moving between Languages & Cultural Frameworks, eds. Su Kim Lee, Siew Ming Thang, and King Siong Lee (Subang Jaya, Selangor, Malaysia: Pelanduk Publications, 2007), 7.
60See, for example, Prashanth Parameswaran, “Southeast Asia and US-China Competition: Contours, Realities, and Implications for the Indo-Pacific,” Wilson Center, December 21, 2023, https://www.wilsoncenter.org/article/southeast-asia-and-us-china-competition-contours-realities-and-implications-indo-pacific; and “Southeast Asia in a World of Strategic Competition: An Essay Series,” United States Institute of Peace, September 2023, https://www.usip.org/programs/southeast-asia-world-strategic-competition-essay-series.
61Osmond Chia, “S’pore Aims to Be a Trusted, Neutral Hub for Collaboration in Global AI Race: Chan Chun Sing,” Straits Times, October 7, 2024, https://www.straitstimes.com/singapore/s-pore-aims-to-be-a-trusted-neutral-hub-for-collaboration-in-global-ai-race-chan-chun-sing.
62“AI Singapore,” https://aisingapore.org/, accessed November 29, 2024.
63“Singapore Pioneers S$70m Flagship AI Initiative to Develop Southeast Asia’s First Large Language Model Ecosystem Catering to the Region’s Diverse Culture and Languages,” Singapore Infocomm Media Development Authority, December 4, 2023, https://www.imda.gov.sg/resources/press-releases-factsheets-and-speeches/press-releases/2023/sg-to-develop-southeast-asias-first-llm-ecosystem.
64Chia, “S’pore Aims to Be a Trusted, Neutral Hub for Collaboration in Global AI Race.”
65“ASEAN Investment Report 2024: ASEAN Economic Community 2025 and Foreign Direct Investment,” ASEAN Secretariat and UN Trade and Development, October 9, 2024, https://unctad.org/publication/asean-investment-report-2024.
66“The Structure of ASEAN Economy,” ASEANstats, January 2024, https://www.aseanstats.org/publication/asean-statistical-brief-vol-4-january-2024/.
67“E-Conomy SEA 2024: Profits on the Rise, Harnessing SEA’s Advantage,” Google, Temasek, Bain & Company, November 2024, https://services.google.com/fh/files/misc/e_conomy_sea_2024_report.pdf.
68Yogesh Hirdaramani, “How ASEAN’s First Large Language Model Will Support Businesses,” GovInsider, February 23, 2024, https://govinsider.asia/intl-en/article/how-aseans-first-large-language-model-will-support-businesses.
69“If You Don’t Understand, How Can You Learn?,” Global Education Monitoring Report: Policy Paper, 24, United Nations Education, Scientific and Cultural Organization, February 2016, https://unesdoc.unesco.org/ark:/48223/pf0000243713.
70Suwilai Premsrirat and Mirinda Burarungrot, “Multilingualism, Bi/Multilingual Education and Social Inclusion: A Case Study in Southern Thailand,” Manusya: Journal of Humanities 24, no. 3 (2022): 373–89, https://doi.org/10.1163/26659077-24030006.
71Stephen L. Walter and Carol Benson, “Language Policy and Medium of Instruction in Formal Education,” in The Cambridge Handbook of Language Policy, ed. Bernard Spolsky, Cambridge Handbooks in Language and Linguistics (Cambridge: Cambridge University Press, 2012), 278–300, https://doi.org/10.1017/CBO9780511979026.017.
72“If You Don’t Understand, How Can You Learn?”
73Kimmo Kosonen, “Language-in-Education Policies in Southeast Asia: An Overview,” in Mother Tongue as Bridge Language of Instruction: Policies and Experiences in Southeast Asia, eds. Kimmo Kosonen and Catherine Young (Bangkok: Southeast Asian Ministers of Education Organization, World Bank, and Education for All, 2009), 22–43.
74Bernama News, “MBMBI to Be Implemented One Year Earlier Than Planned-Muhyiddin,” 2009; and Sarah Kaur Gill and Azianura Hani Shaari, “Malaysia’s Complex Language Policy Journey via Bahasa Melayu and English,” in The Routledge International Handbook of Language Education Policy in Asia, by Andy Kirkpatrick and Anthony J. Liddicoat, eds. Andy Kirkpatrick and Anthony J. Liddicoat, 1st ed. (Abingdon, Oxon; NY: Routledge, 2019), 257–71, https://doi.org/10.4324/9781315666235.
75“If You Don’t Understand, How Can You Learn?”
76Mikihiro Moriyama, “Regional Languages and Decentralisation in Post-New Order Indonesia: The Case of Sundanese,” in Words in Motion: Language and Discourse in Post-New Order Indonesia, eds. Keith Foulcher, Mikihiro Moriyama, and Manneke Budiman (Singapore: National University of Singapore Press, 2012), 82–100; George Quinn, “Emerging from Dire Straits: Post-New Order Developments in Javanese Language and Literature,” in Words in Motion: Language and Discourse in Post-New Order Indonesia, eds. Keith Foulcher, Mikihiro Moriyama, and Manneke Budiman (Singapore: National University of Singapore Press, 2012), 65–81; and Kohler, “Language Education Policy in Indonesia,” 289.
77Truong et al., “Crossing Linguistic Horizons.”
78Alham Fikri Aji, Genta Indra Winata, Fajri Koto, Samuel Cahyawijaya, Ade Romadhony, et al., “One Country, 700+ Languages: NLP Challenges for Underrepresented Languages and Dialects in Indonesia,” Proceedings of the 60th Annual Meeting of the Association for Computational Linguistics, Association for Computational Linguistics, May 2022, 7226–49, https://doi.org/10.18653/v1/2022.acl-long.500; and James Vo, “Vi-Mistral-X: Building a Vietnamese Language Model With Advanced Continual Pre-Training,” arXiv, March 20, 2024, https://doi.org/10.48550/arXiv.2403.15470.
79Eberhard, Simons, and Fennig, Ethnologue: Languages of the World.
80Abigail C. Cohn and Maya Ravindranath, “Local Languages in Indonesia: Language Maintenance or Language Shift?,” Linguistik Indonesia 32, no. 2 (2014): 131–48, https://doi.org/10.26499/li.v32i2.22.
81Samuel Cahyawijaya, Holy Lovenia, Fajri Koto, Rifki Afina Putri, Emmanuel Dave, et al., “Cendol: Open Instruction-Tuned Generative Large Language Models for Indonesian Languages,” arXiv, July 7, 2024, https://doi.org/10.48550/arXiv.2404.06138.
82Cahyawijaya et al., “Cendol”; and Orevaoghene Ahia, Sachin Kumar, Hila Gonen, Jungo Kasai, David Mortensen, et al., “Do All Languages Cost the Same? Tokenization in the Era of Commercial Language Models,” Proceedings of the 2023 Conference on Empirical Methods in Natural Language Processing, Association for Computational Linguistics, 2023, 9904–23, https://doi.org/10.18653/v1/2023.emnlp-main.614.
83Ahia et al., “Do All Languages Cost the Same?”
84Osmond Chia, “AI Masters Singlish in Key Breakthrough to Serve Healthcare and Patients’ Needs,” Straits Times, November 14, 2024, https://www.straitstimes.com/singapore/ai-masters-singlish-in-key-breakthrough-to-serve-healthcare-and-patients-needs.
85Dipam Chakraborty, “Fine Tuning the H2O Danube2 LLM for the Singlish Language,” H2O.Ai (blog), June 3, 2024, https://h2o.ai/blog/2024/Fine-Tuning-the-H2O-Danube2-LLM-for-the-Singlish-language/; and Lynnette Hui Xian Ng and Luo Qi Chan, “Limpeh Ga Li Gong: Challenges in Singlish Annotations,” arXiv, October 21, 2024, https://doi.org/10.48550/arXiv.2410.16156.
86Author in-person interviews with academics and NLP researchers, Singapore and Indonesia, July 2024.
87Chakraborty, “Fine Tuning The H2O Danube2 LLM for The Singlish Language.”
88“Discover Indonesia’s Linguistic Diversity: FAIR Forward and Prosa.Ai on the Road to Inclusive AI Technology,” BMZ Digital.Global, January 16, 2024, https://www.bmz-digital.global/en/entdeckung-der-sprachlichen-vielfalt-indonesiens-fair-forward-und-prosa-ai-auf-dem-weg-zu-inklusiver-ki-sprachtechnologie/.
89Adrian Vickers, “Balinese Texts and Historiography,” History and Theory 29, no. 2 (1990): 158–68, https://doi.org/10.2307/2505223.
90Ibid.
91See, for example, Thanachate Wisaijorn, Space and Time in Thai-Lao Relations: Borderlands in International Relations, 1st Edition (London: Routledge, Taylor & Francis Group, 2022).
92Carl Mika, “Stuck in Time and Space? Thinking through the Māori Term ‘Wā,’” Atlántica 2, 2021, 23–30, https://www.revistaatlantica.com/en/stuck-in-time-and-space-thinking-through-the-maori-term-wa-2/.
93“Māori & Pākehā Conceptualisations of Time,” The Hour Glass, March 2, 2020, https://www.thehourglass.com/cultural-perspectives/maori-time/.
94Anthony Milner, “Nama, Group-Binding and Moral Balance: Themes and Origins of Malaysian Foreign Policy,” Institute of Strategic and International Studies Malaysia, 2015, https://isis.org.my/wp-content/uploads/2015/09/attachments_e-books_Milner_Monograph_2015.pdf.
95Clive Kessler, “Daulat, Kedaulatan, Sovereignty and Constitutionalism,” New Mandala, March 3, 2014, https://www.newmandala.org/daulat-kedaulatan-sovereignty-and-constitutionalism/.
96Andrew Smart, Ben Hutchinson, Lameck Mbangula Amugongo, Suzanne Dikker, Alex Zito, et al., “Socially Responsible Data for Large Multilingual Language Models,” arXiv, September 8, 2024, https://doi.org/10.48550/arXiv.2409.05247.
97Jay Ke-Schutte, Angloscene: Compromised Personhood in Afro-Chinese Translations (California: University of California Press, 2023).
98Angella Ndaka and Geri Karuri-Sebina, “Whose Agenda Is Being Served by the Dynamics Surrounding AI African Data Extraction?,” Daily Maverick, April 22, 2024, https://www.dailymaverick.co.za/article/2024-04-22-whose-agenda-is-being-served-by-the-dynamics-surrounding-ai-african-data-extraction/.
99Smart et al., “Socially Responsible Data for Large Multilingual Language Models.”
100See, for example, “Behind the AI Curtain: The Invisible Workers Powering AI Development,” International Loabour Organization, February 29, 2024, https://www.ilo.org/meetings-and-events/behind-ai-curtain-invisible-workers-powering-ai-development.
101Salvaggio, “A Meta Analysis.”
102“A Collective Voice for Responsible and Open AI: AfricAI & the Africa-Asia AI Policymaker Network,” BMZ Digital.Global, August 15, 2023, https://www.bmz-digital.global/en/eine-kollektive-stimme-fuer-verantwortungsvolle-und-offene-ki-africai-das-africa-asia-ai-policymaker-network/.
103“Home,” Te Mana Raraunga Māori Data Sovereignty, accessed December 6, 2024, https://www.temanararaunga.maori.nz.
104Abdullahi Tsanniarchive, “What Africa Needs to Do to Become a Major AI Player,” MIT Technology Review, November 11, 2024, https://www.technologyreview.com/2024/11/11/1106762/africa-ai-barriers/.
105 Fourteenth Parliament of Singapore – Second Session for the Sitting on 10 January 2024.
“National Multimodal Large Language Model Programme (PQ Reply by Minister Josephine Teo): Use of Non-textual Training Data for Singapore's Common Languages in National Multimodal Large Language Model Programme,” SmartNation Singapore, January 10, 2024, https://www.smartnation.gov.sg/media-hub/parliament/10012024b/.
106Tsanniarchive, “What Africa Needs to Do to Become a Major AI Player.”
107Emily M. Bender, Timnit Gebru, Angelina McMillan-Major, and Shmargaret Shmitchell, “On the Dangers of Stochastic Parrots: Can Language Models Be Too Big?,” Proceedings of the 2021 ACM Conference on Fairness, Accountability, and Transparency, Association for Computing Machinery, 2021, 610–23, https://doi.org/10.1145/3442188.3445922.
108Bender et al., “On the Dangers of Stochastic Parrots.”
109Wang et al., “History, Development, and Principles of Large Language Models.”
110Wayne Xin Zhao, Kun Zhou, Junyi Li, Tianyi Tang, Xiaolei Wang, et al., “A Survey of Large Language Models,” arXiv, October 13, 2024, https://doi.org/10.48550/arXiv.2303.18223.
111Ibid.
112Ibid.
113Juan Luis Gastaldi, John Terilla, Luca Malagutti, Brian DuSell, Tim Vieira, et al., “The Foundations of Tokenization: Statistical and Computational Concerns,” arXiv, November 4, 2024, https://doi.org/10.48550/arXiv.2407.11606.
114Humza Naveed, Asad Ullah Khan, Shi Qiu, Muhammad Saqib, Saeed Anwar, et al., “A Comprehensive Overview of Large Language Models,” arXiv, October 17, 2024, https://doi.org/10.48550/arXiv.2307.06435.
115Zhao et al., “A Survey of Large Language Models.”
116Venkatesh Balavadhani Parthasarathy, Ahtsham Zafar, Aafaq Khan, and Arsalan Shahid, “The Ultimate Guide to Fine-Tuning LLMs from Basics to Breakthroughs: An Exhaustive Review of Technologies, Research, Best Practices, Applied Research Challenges and Opportunities,” arXiv, October 30, 2024, https://doi.org/10.48550/arXiv.2408.13296.
117Yupeng Chang et al., “A Survey on Evaluation of Large Language Models” (arXiv, December 29, 2023), https://doi.org/10.48550/arXiv.2307.03109.
118Michael Goodwin, “API: The Key to Modern Business Innovation,” International Business Machines Corporation (IBM), April 9, 2024, https://www.ibm.com/think/topics/api.